Changes:
- Disclaimer in README
- Replaced all occurences of Gym with Gymnasium
- Removed code that is now dead since we no longer need to support the
old step API
- Updated type hints to only allow new step API
- Increased required version of envpool to support Gymnasium
- Increased required version of PettingZoo to support Gymnasium
- Updated `PettingZooEnv` to only use the new step API, removed hack to
also support old API
- I had to add some `# type: ignore` comments, due to new type hinting
in Gymnasium. I'm not that familiar with type hinting but I believe that
the issue is on the Gymnasium side and we are looking into it.
- Had to update `MyTestEnv` to support `options` kwarg
- Skip NNI tests because they still use OpenAI Gym
- Also allow `PettingZooEnv` in vector environment
- Updated doc page about ReplayBuffer to also talk about terminated and
truncated flags.
Still need to do:
- Update the Jupyter notebooks in docs
- Check the entire code base for more dead code (from compatibility
stuff)
- Check the reset functions of all environments/wrappers in code base to
make sure they use the `options` kwarg
- Someone might want to check test_env_finite.py
- Is it okay to allow `PettingZooEnv` in vector environments? Might need
to update docs?
This PR addresses #772 (updates Atari wrappers to work with new Gym API)
and some additional issues:
- Pre-commit was using gitlab for flake8, which as of recently requires
authentication -> Replaced with GitHub
- Yapf was quietly failing in pre-commit. Changed it such that it fixes
formatting in-place
- There is an incompatibility between flake8 and yapf where yapf puts
binary operators after the line break and flake8 wants it before the
break. I added an exception for flake8.
- Also require `packaging` in setup.py
My changes shouldn't change the behaviour of the wrappers for older
versions, but please double check.
Idk whether it's just me, but there are always some incompatibilities
between yapf and flake8 that need to resolved manually. It might make
sense to try black instead.
- [x] I have marked all applicable categories:
+ [ ] exception-raising fix
+ [x] algorithm implementation fix
+ [ ] documentation modification
+ [ ] new feature
- [x] I have reformatted the code using `make format` (**required**)
- [x] I have checked the code using `make commit-checks` (**required**)
- [x] If applicable, I have mentioned the relevant/related issue(s)
- [x] If applicable, I have listed every items in this Pull Request
below
While trying to debug Atari PPO+LSTM, I found significant gap between
our Atari PPO example vs [CleanRL's Atari PPO w/
EnvPool](https://docs.cleanrl.dev/rl-algorithms/ppo/#ppo_atari_envpoolpy).
I tried to align our implementation with CleaRL's version, mostly in
hyper parameter choices, and got significant gain in Breakout, Qbert,
SpaceInvaders while on par in other games. After this fix, I would
suggest updating our [Atari
Benchmark](https://tianshou.readthedocs.io/en/master/tutorials/benchmark.html)
PPO experiments.
A few interesting findings:
- Layer initialization helps stabilize the training and enable the use
of larger learning rates; without it, larger learning rates will trigger
NaN gradient very quickly;
- ppo.py#L97-L101: this change helps training stability for reasons I do
not understand; also it makes the GPU usage higher.
Shoutout to [CleanRL](https://github.com/vwxyzjn/cleanrl) for a
well-tuned Atari PPO reference implementation!
## implementation
I implemented HER solely as a replay buffer. It is done by temporarily
directly re-writing transitions storage (`self._meta`) during the
`sample_indices()` call. The original transitions are cached and will be
restored at the beginning of the next sampling or when other methods is
called. This will make sure that. for example, n-step return calculation
can be done without altering the policy.
There is also a problem with the original indices sampling. The sampled
indices are not guaranteed to be from different episodes. So I decided
to perform re-writing based on the episode. This guarantees that the
sampled transitions from the same episode will have the same re-written
goal. This also make the re-writing ratio calculation slightly differ
from the paper, but it won't be too different if there are many episodes
in the buffer.
In the current commit, HER replay buffer only support 'future' strategy
and online sampling. This is the best of HER in term of performance and
memory efficiency.
I also add a few more convenient replay buffers
(`HERVectorReplayBuffer`, `HERReplayBufferManager`), test env
(`MyGoalEnv`), gym wrapper (`TruncatedAsTerminated`), unit tests, and a
simple example (examples/offline/fetch_her_ddpg.py).
## verification
I have added unit tests for almost everything I have implemented.
HER replay buffer was also tested using DDPG on [`FetchReach-v3`
env](https://github.com/Farama-Foundation/Gymnasium-Robotics). I used
default DDPG parameters from mujoco example and didn't tune anything
further to get this good result! (train script:
examples/offline/fetch_her_ddpg.py).
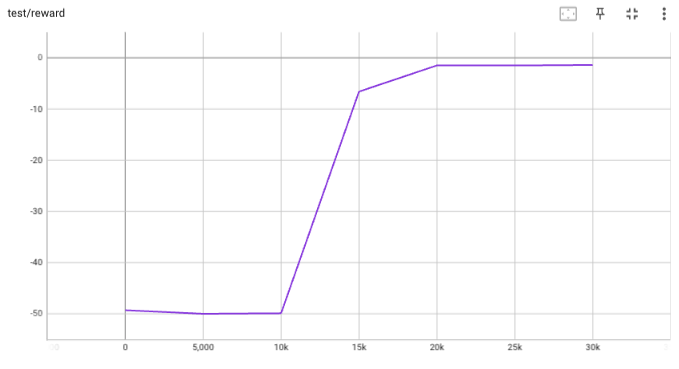
fixes some deprecation warnings due to new changes in gym version 0.23:
- use `env.np_random.integers` instead of `env.np_random.randint`
- support `seed` and `return_info` arguments for reset (addresses https://github.com/thu-ml/tianshou/issues/605)
- A DummyTqdm class added to utils: it replicates the interface used by trainers, but does not show the progress bar;
- Added a show_progress argument to the base trainer: when show_progress == True, dummy_tqdm is used in place of tqdm.
add imitation baselines for offline RL; make the choice of env/task and D4RL dataset explicit; on expert datasets, IL easily outperforms; after reading the D4RL paper, I'll rerun the exps on medium data
* Use `global_step` as the x-axis for wandb
* Use Tensorboard SummaryWritter as core with `wandb.init(..., sync_tensorboard=True)`
* Update all atari examples with wandb
Co-authored-by: Jiayi Weng <trinkle23897@gmail.com>
- Fixes an inconsistency in the implementation of Discrete CRR. Now it uses `Critic` class for its critic, following conventions in other actor-critic policies;
- Updates several offline policies to use `ActorCritic` class for its optimizer to eliminate randomness caused by parameter sharing between actor and critic;
- Add `writer.flush()` in TensorboardLogger to ensure real-time result;
- Enable `test_collector=None` in 3 trainers to turn off testing during training;
- Updates the Atari offline results in README.md;
- Moves Atari offline RL examples to `examples/offline`; tests to `test/offline` per review comments.
This PR implements BCQPolicy, which could be used to train an offline agent in the environment of continuous action space. An experimental result 'halfcheetah-expert-v1' is provided, which is a d4rl environment (for Offline Reinforcement Learning).
Example usage is in the examples/offline/offline_bcq.py.