Initial Commit
This commit is contained in:
commit
fb5c21557a
134
.gitignore
vendored
Normal file
134
.gitignore
vendored
Normal file
@ -0,0 +1,134 @@
|
||||
#
|
||||
*.sh
|
||||
logdir*
|
||||
vis_*
|
||||
|
||||
# Byte-compiled / optimized / DLL files
|
||||
__pycache__/
|
||||
*.py[cod]
|
||||
*$py.class
|
||||
|
||||
# C extensions
|
||||
*.so
|
||||
|
||||
# Distribution / packaging
|
||||
.Python
|
||||
build/
|
||||
develop-eggs/
|
||||
dist/
|
||||
downloads/
|
||||
eggs/
|
||||
.eggs/
|
||||
lib/
|
||||
lib64/
|
||||
parts/
|
||||
sdist/
|
||||
var/
|
||||
wheels/
|
||||
pip-wheel-metadata/
|
||||
share/python-wheels/
|
||||
*.egg-info/
|
||||
.installed.cfg
|
||||
*.egg
|
||||
MANIFEST
|
||||
|
||||
# PyInstaller
|
||||
# Usually these files are written by a python script from a template
|
||||
# before PyInstaller builds the exe, so as to inject date/other infos into it.
|
||||
*.manifest
|
||||
*.spec
|
||||
|
||||
# Installer logs
|
||||
pip-log.txt
|
||||
pip-delete-this-directory.txt
|
||||
|
||||
# Unit test / coverage reports
|
||||
htmlcov/
|
||||
.tox/
|
||||
.nox/
|
||||
.coverage
|
||||
.coverage.*
|
||||
.cache
|
||||
nosetests.xml
|
||||
coverage.xml
|
||||
*.cover
|
||||
*.py,cover
|
||||
.hypothesis/
|
||||
.pytest_cache/
|
||||
|
||||
# Translations
|
||||
*.mo
|
||||
*.pot
|
||||
|
||||
# Django stuff:
|
||||
*.log
|
||||
local_settings.py
|
||||
db.sqlite3
|
||||
db.sqlite3-journal
|
||||
|
||||
# Flask stuff:
|
||||
instance/
|
||||
.webassets-cache
|
||||
|
||||
# Scrapy stuff:
|
||||
.scrapy
|
||||
|
||||
# Sphinx documentation
|
||||
docs/_build/
|
||||
|
||||
# PyBuilder
|
||||
target/
|
||||
|
||||
# Jupyter Notebook
|
||||
.ipynb_checkpoints
|
||||
|
||||
# IPython
|
||||
profile_default/
|
||||
ipython_config.py
|
||||
|
||||
# pyenv
|
||||
.python-version
|
||||
|
||||
# pipenv
|
||||
# According to pypa/pipenv#598, it is recommended to include Pipfile.lock in version control.
|
||||
# However, in case of collaboration, if having platform-specific dependencies or dependencies
|
||||
# having no cross-platform support, pipenv may install dependencies that don't work, or not
|
||||
# install all needed dependencies.
|
||||
#Pipfile.lock
|
||||
|
||||
# PEP 582; used by e.g. github.com/David-OConnor/pyflow
|
||||
__pypackages__/
|
||||
|
||||
# Celery stuff
|
||||
celerybeat-schedule
|
||||
celerybeat.pid
|
||||
|
||||
# SageMath parsed files
|
||||
*.sage.py
|
||||
|
||||
# Environments
|
||||
.env
|
||||
.venv
|
||||
env/
|
||||
venv/
|
||||
ENV/
|
||||
env.bak/
|
||||
venv.bak/
|
||||
|
||||
# Spyder project settings
|
||||
.spyderproject
|
||||
.spyproject
|
||||
|
||||
# Rope project settings
|
||||
.ropeproject
|
||||
|
||||
# mkdocs documentation
|
||||
/site
|
||||
|
||||
# mypy
|
||||
.mypy_cache/
|
||||
.dmypy.json
|
||||
dmypy.json
|
||||
|
||||
# Pyre type checker
|
||||
.pyre/
|
21
LICENSE
Normal file
21
LICENSE
Normal file
@ -0,0 +1,21 @@
|
||||
MIT License
|
||||
|
||||
Copyright (c) 2023 NM512
|
||||
|
||||
Permission is hereby granted, free of charge, to any person obtaining a copy
|
||||
of this software and associated documentation files (the "Software"), to deal
|
||||
in the Software without restriction, including without limitation the rights
|
||||
to use, copy, modify, merge, publish, distribute, sublicense, and/or sell
|
||||
copies of the Software, and to permit persons to whom the Software is
|
||||
furnished to do so, subject to the following conditions:
|
||||
|
||||
The above copyright notice and this permission notice shall be included in all
|
||||
copies or substantial portions of the Software.
|
||||
|
||||
THE SOFTWARE IS PROVIDED "AS IS", WITHOUT WARRANTY OF ANY KIND, EXPRESS OR
|
||||
IMPLIED, INCLUDING BUT NOT LIMITED TO THE WARRANTIES OF MERCHANTABILITY,
|
||||
FITNESS FOR A PARTICULAR PURPOSE AND NONINFRINGEMENT. IN NO EVENT SHALL THE
|
||||
AUTHORS OR COPYRIGHT HOLDERS BE LIABLE FOR ANY CLAIM, DAMAGES OR OTHER
|
||||
LIABILITY, WHETHER IN AN ACTION OF CONTRACT, TORT OR OTHERWISE, ARISING FROM,
|
||||
OUT OF OR IN CONNECTION WITH THE SOFTWARE OR THE USE OR OTHER DEALINGS IN THE
|
||||
SOFTWARE.
|
33
README.md
Normal file
33
README.md
Normal file
@ -0,0 +1,33 @@
|
||||
# Dreamer-v3 Pytorch
|
||||
Pytorch implementation of [Mastering Diverse Domains through World Models](https://arxiv.org/abs/2301.04104v1)
|
||||
|
||||
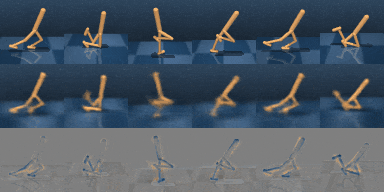
|
||||
|
||||
## Instructions
|
||||
Get dependencies:
|
||||
```
|
||||
pip install -r requirements.txt
|
||||
```
|
||||
Train the agent:
|
||||
```
|
||||
python3 dreamer.py --configs defaults --logdir $ABSOLUTEPATH_TO_SAVE_LOG
|
||||
```
|
||||
Monitor results:
|
||||
```
|
||||
tensorboard --logdir $ABSOLUTEPATH_TO_SAVE_LOG
|
||||
```
|
||||
## Evaluation Results
|
||||
work-in-progress
|
||||
|
||||
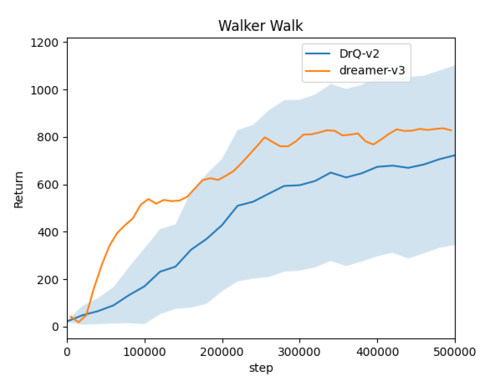
|
||||
|
||||
## Awesome Environments used for testing:
|
||||
- Deepmind control suite: https://github.com/deepmind/dm_control
|
||||
- will be added soon
|
||||
|
||||
## Acknowledgments
|
||||
This code is heavily inspired by the following works:
|
||||
- danijar's Dreamer-v2 tensorflow implementation: https://github.com/danijar/dreamerv2
|
||||
- jsikyoon's Dreamer-v2 pytorch implementation: https://github.com/jsikyoon/dreamer-torch
|
||||
- RajGhugare19's Dreamer-v2 pytorch implementation: https://github.com/RajGhugare19/dreamerv2
|
||||
- denisyarats's DrQ-v2 original implementation: https://github.com/facebookresearch/drqv2
|
136
configs.yaml
Normal file
136
configs.yaml
Normal file
@ -0,0 +1,136 @@
|
||||
defaults:
|
||||
|
||||
logdir: null
|
||||
traindir: null
|
||||
evaldir: null
|
||||
offline_traindir: ''
|
||||
offline_evaldir: ''
|
||||
seed: 0
|
||||
steps: 5e5
|
||||
eval_every: 1e4
|
||||
log_every: 1e4
|
||||
reset_every: 0
|
||||
#gpu_growth: True
|
||||
device: 'cuda:0'
|
||||
precision: 16
|
||||
debug: False
|
||||
expl_gifs: False
|
||||
|
||||
# Environment
|
||||
task: 'dmc_walker_walk'
|
||||
size: [64, 64]
|
||||
envs: 1
|
||||
action_repeat: 2
|
||||
time_limit: 1000
|
||||
grayscale: False
|
||||
prefill: 2500
|
||||
eval_noise: 0.0
|
||||
reward_trans: 'symlog'
|
||||
obs_trans: 'normalize'
|
||||
critic_trans: 'symlog'
|
||||
reward_EMA: True
|
||||
|
||||
# Model
|
||||
dyn_cell: 'gru_layer_norm'
|
||||
dyn_hidden: 512
|
||||
dyn_deter: 512
|
||||
dyn_stoch: 32
|
||||
dyn_discrete: 32
|
||||
dyn_input_layers: 1
|
||||
dyn_output_layers: 1
|
||||
dyn_rec_depth: 1
|
||||
dyn_shared: False
|
||||
dyn_mean_act: 'none'
|
||||
dyn_std_act: 'sigmoid2'
|
||||
dyn_min_std: 0.1
|
||||
dyn_temp_post: True
|
||||
grad_heads: ['image', 'reward', 'discount']
|
||||
units: 256
|
||||
reward_layers: 2
|
||||
discount_layers: 2
|
||||
value_layers: 2
|
||||
actor_layers: 2
|
||||
act: 'SiLU'
|
||||
norm: 'LayerNorm'
|
||||
cnn_depth: 32
|
||||
encoder_kernels: [3, 3, 3, 3]
|
||||
decoder_kernels: [3, 3, 3, 3]
|
||||
# changed here
|
||||
value_head: 'twohot'
|
||||
reward_head: 'twohot'
|
||||
kl_lscale: '0.1'
|
||||
kl_rscale: '0.5'
|
||||
kl_free: '1.0'
|
||||
kl_forward: False
|
||||
pred_discount: True
|
||||
discount_scale: 1.0
|
||||
reward_scale: 1.0
|
||||
weight_decay: 0.0
|
||||
unimix_ratio: 0.01
|
||||
|
||||
# Training
|
||||
batch_size: 16
|
||||
batch_length: 64
|
||||
train_every: 5
|
||||
train_steps: 1
|
||||
pretrain: 100
|
||||
model_lr: 1e-4
|
||||
opt_eps: 1e-8
|
||||
grad_clip: 1000
|
||||
value_lr: 3e-5
|
||||
actor_lr: 3e-5
|
||||
ac_opt_eps: 1e-5
|
||||
value_grad_clip: 100
|
||||
actor_grad_clip: 100
|
||||
dataset_size: 0
|
||||
oversample_ends: False
|
||||
slow_value_target: True
|
||||
slow_actor_target: True
|
||||
slow_target_update: 50
|
||||
slow_target_fraction: 0.01
|
||||
opt: 'adam'
|
||||
|
||||
# Behavior.
|
||||
discount: 0.997
|
||||
discount_lambda: 0.95
|
||||
imag_horizon: 15
|
||||
imag_gradient: 'dynamics'
|
||||
imag_gradient_mix: '0.1'
|
||||
imag_sample: True
|
||||
actor_dist: 'trunc_normal'
|
||||
actor_entropy: '3e-4'
|
||||
actor_state_entropy: 0.0
|
||||
actor_init_std: 1.0
|
||||
actor_min_std: 0.1
|
||||
actor_disc: 5
|
||||
actor_temp: 0.1
|
||||
actor_outscale: 0.0
|
||||
expl_amount: 0.0
|
||||
eval_state_mean: False
|
||||
collect_dyn_sample: True
|
||||
behavior_stop_grad: True
|
||||
value_decay: 0.0
|
||||
future_entropy: False
|
||||
|
||||
# Exploration
|
||||
expl_behavior: 'greedy'
|
||||
expl_until: 0
|
||||
expl_extr_scale: 0.0
|
||||
expl_intr_scale: 1.0
|
||||
disag_target: 'stoch'
|
||||
disag_log: True
|
||||
disag_models: 10
|
||||
disag_offset: 1
|
||||
disag_layers: 4
|
||||
disag_units: 400
|
||||
disag_action_cond: False
|
||||
|
||||
debug:
|
||||
|
||||
debug: True
|
||||
pretrain: 1
|
||||
prefill: 1
|
||||
train_steps: 1
|
||||
batch_size: 10
|
||||
batch_length: 20
|
||||
|
343
dreamer.py
Normal file
343
dreamer.py
Normal file
@ -0,0 +1,343 @@
|
||||
import argparse
|
||||
import collections
|
||||
import functools
|
||||
import os
|
||||
import pathlib
|
||||
import sys
|
||||
import warnings
|
||||
|
||||
os.environ["MUJOCO_GL"] = "egl"
|
||||
|
||||
import numpy as np
|
||||
import ruamel.yaml as yaml
|
||||
|
||||
sys.path.append(str(pathlib.Path(__file__).parent))
|
||||
|
||||
import exploration as expl
|
||||
import models
|
||||
import tools
|
||||
import wrappers
|
||||
|
||||
import torch
|
||||
from torch import nn
|
||||
from torch import distributions as torchd
|
||||
|
||||
to_np = lambda x: x.detach().cpu().numpy()
|
||||
|
||||
|
||||
class Dreamer(nn.Module):
|
||||
def __init__(self, config, logger, dataset):
|
||||
super(Dreamer, self).__init__()
|
||||
self._config = config
|
||||
self._logger = logger
|
||||
self._should_log = tools.Every(config.log_every)
|
||||
self._should_train = tools.Every(config.train_every)
|
||||
self._should_pretrain = tools.Once()
|
||||
self._should_reset = tools.Every(config.reset_every)
|
||||
self._should_expl = tools.Until(int(config.expl_until / config.action_repeat))
|
||||
self._metrics = {}
|
||||
self._step = count_steps(config.traindir)
|
||||
# Schedules.
|
||||
config.actor_entropy = lambda x=config.actor_entropy: tools.schedule(
|
||||
x, self._step
|
||||
)
|
||||
config.actor_state_entropy = (
|
||||
lambda x=config.actor_state_entropy: tools.schedule(x, self._step)
|
||||
)
|
||||
config.imag_gradient_mix = lambda x=config.imag_gradient_mix: tools.schedule(
|
||||
x, self._step
|
||||
)
|
||||
self._dataset = dataset
|
||||
self._wm = models.WorldModel(self._step, config)
|
||||
self._task_behavior = models.ImagBehavior(
|
||||
config, self._wm, config.behavior_stop_grad
|
||||
)
|
||||
reward = lambda f, s, a: self._wm.heads["reward"](f).mean
|
||||
self._expl_behavior = dict(
|
||||
greedy=lambda: self._task_behavior,
|
||||
random=lambda: expl.Random(config),
|
||||
plan2explore=lambda: expl.Plan2Explore(config, self._wm, reward),
|
||||
)[config.expl_behavior]()
|
||||
|
||||
def __call__(self, obs, reset, state=None, reward=None, training=True):
|
||||
step = self._step
|
||||
if self._should_reset(step):
|
||||
state = None
|
||||
if state is not None and reset.any():
|
||||
mask = 1 - reset
|
||||
for key in state[0].keys():
|
||||
for i in range(state[0][key].shape[0]):
|
||||
state[0][key][i] *= mask[i]
|
||||
for i in range(len(state[1])):
|
||||
state[1][i] *= mask[i]
|
||||
if training and self._should_train(step):
|
||||
steps = (
|
||||
self._config.pretrain
|
||||
if self._should_pretrain()
|
||||
else self._config.train_steps
|
||||
)
|
||||
for _ in range(steps):
|
||||
self._train(next(self._dataset))
|
||||
if self._should_log(step):
|
||||
for name, values in self._metrics.items():
|
||||
self._logger.scalar(name, float(np.mean(values)))
|
||||
self._metrics[name] = []
|
||||
openl = self._wm.video_pred(next(self._dataset))
|
||||
self._logger.video("train_openl", to_np(openl))
|
||||
self._logger.write(fps=True)
|
||||
|
||||
policy_output, state = self._policy(obs, state, training)
|
||||
|
||||
if training:
|
||||
self._step += len(reset)
|
||||
self._logger.step = self._config.action_repeat * self._step
|
||||
return policy_output, state
|
||||
|
||||
def _policy(self, obs, state, training):
|
||||
if state is None:
|
||||
batch_size = len(obs["image"])
|
||||
latent = self._wm.dynamics.initial(len(obs["image"]))
|
||||
action = torch.zeros((batch_size, self._config.num_actions)).to(
|
||||
self._config.device
|
||||
)
|
||||
else:
|
||||
latent, action = state
|
||||
embed = self._wm.encoder(self._wm.preprocess(obs))
|
||||
latent, _ = self._wm.dynamics.obs_step(
|
||||
latent, action, embed, self._config.collect_dyn_sample
|
||||
)
|
||||
if self._config.eval_state_mean:
|
||||
latent["stoch"] = latent["mean"]
|
||||
feat = self._wm.dynamics.get_feat(latent)
|
||||
if not training:
|
||||
actor = self._task_behavior.actor(feat)
|
||||
action = actor.mode()
|
||||
elif self._should_expl(self._step):
|
||||
actor = self._expl_behavior.actor(feat)
|
||||
action = actor.sample()
|
||||
else:
|
||||
actor = self._task_behavior.actor(feat)
|
||||
action = actor.sample()
|
||||
logprob = actor.log_prob(action)
|
||||
latent = {k: v.detach() for k, v in latent.items()}
|
||||
action = action.detach()
|
||||
if self._config.actor_dist == "onehot_gumble":
|
||||
action = torch.one_hot(
|
||||
torch.argmax(action, dim=-1), self._config.num_actions
|
||||
)
|
||||
action = self._exploration(action, training)
|
||||
policy_output = {"action": action, "logprob": logprob}
|
||||
state = (latent, action)
|
||||
return policy_output, state
|
||||
|
||||
def _exploration(self, action, training):
|
||||
amount = self._config.expl_amount if training else self._config.eval_noise
|
||||
if amount == 0:
|
||||
return action
|
||||
if "onehot" in self._config.actor_dist:
|
||||
probs = amount / self._config.num_actions + (1 - amount) * action
|
||||
return tools.OneHotDist(probs=probs).sample()
|
||||
else:
|
||||
return torch.clip(torchd.normal.Normal(action, amount).sample(), -1, 1)
|
||||
raise NotImplementedError(self._config.action_noise)
|
||||
|
||||
def _train(self, data):
|
||||
metrics = {}
|
||||
post, context, mets = self._wm._train(data)
|
||||
metrics.update(mets)
|
||||
start = post
|
||||
if self._config.pred_discount: # Last step could be terminal.
|
||||
start = {k: v[:, :-1] for k, v in post.items()}
|
||||
context = {k: v[:, :-1] for k, v in context.items()}
|
||||
reward = lambda f, s, a: self._wm.heads["reward"](
|
||||
self._wm.dynamics.get_feat(s)
|
||||
).mode()
|
||||
metrics.update(self._task_behavior._train(start, reward)[-1])
|
||||
if self._config.expl_behavior != "greedy":
|
||||
if self._config.pred_discount:
|
||||
data = {k: v[:, :-1] for k, v in data.items()}
|
||||
mets = self._expl_behavior.train(start, context, data)[-1]
|
||||
metrics.update({"expl_" + key: value for key, value in mets.items()})
|
||||
for name, value in metrics.items():
|
||||
if not name in self._metrics.keys():
|
||||
self._metrics[name] = [value]
|
||||
else:
|
||||
self._metrics[name].append(value)
|
||||
|
||||
|
||||
def count_steps(folder):
|
||||
return sum(int(str(n).split("-")[-1][:-4]) - 1 for n in folder.glob("*.npz"))
|
||||
|
||||
|
||||
def make_dataset(episodes, config):
|
||||
generator = tools.sample_episodes(
|
||||
episodes, config.batch_length, config.oversample_ends
|
||||
)
|
||||
dataset = tools.from_generator(generator, config.batch_size)
|
||||
return dataset
|
||||
|
||||
|
||||
def make_env(config, logger, mode, train_eps, eval_eps):
|
||||
suite, task = config.task.split("_", 1)
|
||||
if suite == "dmc":
|
||||
env = wrappers.DeepMindControl(task, config.action_repeat, config.size)
|
||||
env = wrappers.NormalizeActions(env)
|
||||
elif suite == "atari":
|
||||
env = wrappers.Atari(
|
||||
task,
|
||||
config.action_repeat,
|
||||
config.size,
|
||||
grayscale=config.grayscale,
|
||||
life_done=False and ("train" in mode),
|
||||
sticky_actions=True,
|
||||
all_actions=True,
|
||||
)
|
||||
env = wrappers.OneHotAction(env)
|
||||
elif suite == "dmlab":
|
||||
env = wrappers.DeepMindLabyrinth(
|
||||
task, mode if "train" in mode else "test", config.action_repeat
|
||||
)
|
||||
env = wrappers.OneHotAction(env)
|
||||
else:
|
||||
raise NotImplementedError(suite)
|
||||
env = wrappers.TimeLimit(env, config.time_limit)
|
||||
env = wrappers.SelectAction(env, key="action")
|
||||
if (mode == "train") or (mode == "eval"):
|
||||
callbacks = [
|
||||
functools.partial(
|
||||
process_episode, config, logger, mode, train_eps, eval_eps
|
||||
)
|
||||
]
|
||||
env = wrappers.CollectDataset(env, callbacks)
|
||||
env = wrappers.RewardObs(env)
|
||||
return env
|
||||
|
||||
|
||||
def process_episode(config, logger, mode, train_eps, eval_eps, episode):
|
||||
directory = dict(train=config.traindir, eval=config.evaldir)[mode]
|
||||
cache = dict(train=train_eps, eval=eval_eps)[mode]
|
||||
filename = tools.save_episodes(directory, [episode])[0]
|
||||
length = len(episode["reward"]) - 1
|
||||
score = float(episode["reward"].astype(np.float64).sum())
|
||||
video = episode["image"]
|
||||
if mode == "eval":
|
||||
cache.clear()
|
||||
if mode == "train" and config.dataset_size:
|
||||
total = 0
|
||||
for key, ep in reversed(sorted(cache.items(), key=lambda x: x[0])):
|
||||
if total <= config.dataset_size - length:
|
||||
total += len(ep["reward"]) - 1
|
||||
else:
|
||||
del cache[key]
|
||||
logger.scalar("dataset_size", total + length)
|
||||
cache[str(filename)] = episode
|
||||
print(f"{mode.title()} episode has {length} steps and return {score:.1f}.")
|
||||
logger.scalar(f"{mode}_return", score)
|
||||
logger.scalar(f"{mode}_length", length)
|
||||
logger.scalar(f"{mode}_episodes", len(cache))
|
||||
if mode == "eval" or config.expl_gifs:
|
||||
logger.video(f"{mode}_policy", video[None])
|
||||
logger.write()
|
||||
|
||||
|
||||
def main(config):
|
||||
logdir = pathlib.Path(config.logdir).expanduser()
|
||||
config.traindir = config.traindir or logdir / "train_eps"
|
||||
config.evaldir = config.evaldir or logdir / "eval_eps"
|
||||
config.steps //= config.action_repeat
|
||||
config.eval_every //= config.action_repeat
|
||||
config.log_every //= config.action_repeat
|
||||
config.time_limit //= config.action_repeat
|
||||
config.act = getattr(torch.nn, config.act)
|
||||
config.norm = getattr(torch.nn, config.norm)
|
||||
|
||||
print("Logdir", logdir)
|
||||
logdir.mkdir(parents=True, exist_ok=True)
|
||||
config.traindir.mkdir(parents=True, exist_ok=True)
|
||||
config.evaldir.mkdir(parents=True, exist_ok=True)
|
||||
step = count_steps(config.traindir)
|
||||
logger = tools.Logger(logdir, config.action_repeat * step)
|
||||
|
||||
print("Create envs.")
|
||||
if config.offline_traindir:
|
||||
directory = config.offline_traindir.format(**vars(config))
|
||||
else:
|
||||
directory = config.traindir
|
||||
train_eps = tools.load_episodes(directory, limit=config.dataset_size)
|
||||
if config.offline_evaldir:
|
||||
directory = config.offline_evaldir.format(**vars(config))
|
||||
else:
|
||||
directory = config.evaldir
|
||||
eval_eps = tools.load_episodes(directory, limit=1)
|
||||
make = lambda mode: make_env(config, logger, mode, train_eps, eval_eps)
|
||||
train_envs = [make("train") for _ in range(config.envs)]
|
||||
eval_envs = [make("eval") for _ in range(config.envs)]
|
||||
acts = train_envs[0].action_space
|
||||
config.num_actions = acts.n if hasattr(acts, "n") else acts.shape[0]
|
||||
|
||||
if not config.offline_traindir:
|
||||
prefill = max(0, config.prefill - count_steps(config.traindir))
|
||||
print(f"Prefill dataset ({prefill} steps).")
|
||||
if hasattr(acts, "discrete"):
|
||||
random_actor = tools.OneHotDist(
|
||||
torch.zeros_like(torch.Tensor(acts.low))[None]
|
||||
)
|
||||
else:
|
||||
random_actor = torchd.independent.Independent(
|
||||
torchd.uniform.Uniform(
|
||||
torch.Tensor(acts.low)[None], torch.Tensor(acts.high)[None]
|
||||
),
|
||||
1,
|
||||
)
|
||||
|
||||
def random_agent(o, d, s, r):
|
||||
action = random_actor.sample()
|
||||
logprob = random_actor.log_prob(action)
|
||||
return {"action": action, "logprob": logprob}, None
|
||||
|
||||
tools.simulate(random_agent, train_envs, prefill)
|
||||
tools.simulate(random_agent, eval_envs, episodes=1)
|
||||
logger.step = config.action_repeat * count_steps(config.traindir)
|
||||
|
||||
print("Simulate agent.")
|
||||
train_dataset = make_dataset(train_eps, config)
|
||||
eval_dataset = make_dataset(eval_eps, config)
|
||||
agent = Dreamer(config, logger, train_dataset).to(config.device)
|
||||
agent.requires_grad_(requires_grad=False)
|
||||
if (logdir / "latest_model.pt").exists():
|
||||
agent.load_state_dict(torch.load(logdir / "latest_model.pt"))
|
||||
agent._should_pretrain._once = False
|
||||
|
||||
state = None
|
||||
while agent._step < config.steps:
|
||||
logger.write()
|
||||
print("Start evaluation.")
|
||||
video_pred = agent._wm.video_pred(next(eval_dataset))
|
||||
logger.video("eval_openl", to_np(video_pred))
|
||||
eval_policy = functools.partial(agent, training=False)
|
||||
tools.simulate(eval_policy, eval_envs, episodes=1)
|
||||
print("Start training.")
|
||||
state = tools.simulate(agent, train_envs, config.eval_every, state=state)
|
||||
torch.save(agent.state_dict(), logdir / "latest_model.pt")
|
||||
for env in train_envs + eval_envs:
|
||||
try:
|
||||
env.close()
|
||||
except Exception:
|
||||
pass
|
||||
|
||||
|
||||
if __name__ == "__main__":
|
||||
parser = argparse.ArgumentParser()
|
||||
parser.add_argument("--configs", nargs="+", required=True)
|
||||
args, remaining = parser.parse_known_args()
|
||||
configs = yaml.safe_load(
|
||||
(pathlib.Path(sys.argv[0]).parent / "configs.yaml").read_text()
|
||||
)
|
||||
defaults = {}
|
||||
for name in args.configs:
|
||||
defaults.update(configs[name])
|
||||
parser = argparse.ArgumentParser()
|
||||
for key, value in sorted(defaults.items(), key=lambda x: x[0]):
|
||||
arg_type = tools.args_type(value)
|
||||
parser.add_argument(f"--{key}", type=arg_type, default=arg_type(value))
|
||||
main(parser.parse_args(remaining))
|
108
exploration.py
Normal file
108
exploration.py
Normal file
@ -0,0 +1,108 @@
|
||||
import torch
|
||||
from torch import nn
|
||||
from torch import distributions as torchd
|
||||
|
||||
import models
|
||||
import networks
|
||||
import tools
|
||||
|
||||
|
||||
class Random(nn.Module):
|
||||
def __init__(self, config):
|
||||
self._config = config
|
||||
|
||||
def actor(self, feat):
|
||||
shape = feat.shape[:-1] + [self._config.num_actions]
|
||||
if self._config.actor_dist == "onehot":
|
||||
return tools.OneHotDist(torch.zeros(shape))
|
||||
else:
|
||||
ones = torch.ones(shape)
|
||||
return tools.ContDist(torchd.uniform.Uniform(-ones, ones))
|
||||
|
||||
def train(self, start, context):
|
||||
return None, {}
|
||||
|
||||
|
||||
# class Plan2Explore(tools.Module):
|
||||
class Plan2Explore(nn.Module):
|
||||
def __init__(self, config, world_model, reward=None):
|
||||
self._config = config
|
||||
self._reward = reward
|
||||
self._behavior = models.ImagBehavior(config, world_model)
|
||||
self.actor = self._behavior.actor
|
||||
stoch_size = config.dyn_stoch
|
||||
if config.dyn_discrete:
|
||||
stoch_size *= config.dyn_discrete
|
||||
size = {
|
||||
"embed": 32 * config.cnn_depth,
|
||||
"stoch": stoch_size,
|
||||
"deter": config.dyn_deter,
|
||||
"feat": config.dyn_stoch + config.dyn_deter,
|
||||
}[self._config.disag_target]
|
||||
kw = dict(
|
||||
inp_dim=config.dyn_stoch, # pytorch version
|
||||
shape=size,
|
||||
layers=config.disag_layers,
|
||||
units=config.disag_units,
|
||||
act=config.act,
|
||||
)
|
||||
self._networks = [networks.DenseHead(**kw) for _ in range(config.disag_models)]
|
||||
self._opt = tools.optimizer(
|
||||
config.opt,
|
||||
self.parameters(),
|
||||
config.model_lr,
|
||||
config.opt_eps,
|
||||
config.weight_decay,
|
||||
)
|
||||
# self._opt = tools.Optimizer(
|
||||
# 'ensemble', config.model_lr, config.opt_eps, config.grad_clip,
|
||||
# config.weight_decay, opt=config.opt)
|
||||
|
||||
def train(self, start, context, data):
|
||||
metrics = {}
|
||||
stoch = start["stoch"]
|
||||
if self._config.dyn_discrete:
|
||||
stoch = tf.reshape(
|
||||
stoch, stoch.shape[:-2] + (stoch.shape[-2] * stoch.shape[-1])
|
||||
)
|
||||
target = {
|
||||
"embed": context["embed"],
|
||||
"stoch": stoch,
|
||||
"deter": start["deter"],
|
||||
"feat": context["feat"],
|
||||
}[self._config.disag_target]
|
||||
inputs = context["feat"]
|
||||
if self._config.disag_action_cond:
|
||||
inputs = tf.concat([inputs, data["action"]], -1)
|
||||
metrics.update(self._train_ensemble(inputs, target))
|
||||
metrics.update(self._behavior.train(start, self._intrinsic_reward)[-1])
|
||||
return None, metrics
|
||||
|
||||
def _intrinsic_reward(self, feat, state, action):
|
||||
inputs = feat
|
||||
if self._config.disag_action_cond:
|
||||
inputs = tf.concat([inputs, action], -1)
|
||||
preds = [head(inputs, tf.float32).mean() for head in self._networks]
|
||||
disag = tf.reduce_mean(tf.math.reduce_std(preds, 0), -1)
|
||||
if self._config.disag_log:
|
||||
disag = tf.math.log(disag)
|
||||
reward = self._config.expl_intr_scale * disag
|
||||
if self._config.expl_extr_scale:
|
||||
reward += tf.cast(
|
||||
self._config.expl_extr_scale * self._reward(feat, state, action),
|
||||
tf.float32,
|
||||
)
|
||||
return reward
|
||||
|
||||
def _train_ensemble(self, inputs, targets):
|
||||
if self._config.disag_offset:
|
||||
targets = targets[:, self._config.disag_offset :]
|
||||
inputs = inputs[:, : -self._config.disag_offset]
|
||||
targets = tf.stop_gradient(targets)
|
||||
inputs = tf.stop_gradient(inputs)
|
||||
with tf.GradientTape() as tape:
|
||||
preds = [head(inputs) for head in self._networks]
|
||||
likes = [tf.reduce_mean(pred.log_prob(targets)) for pred in preds]
|
||||
loss = -tf.cast(tf.reduce_sum(likes), tf.float32)
|
||||
metrics = self._opt(tape, loss, self._networks)
|
||||
return metrics
|
509
models.py
Normal file
509
models.py
Normal file
@ -0,0 +1,509 @@
|
||||
import copy
|
||||
import torch
|
||||
from torch import nn
|
||||
import numpy as np
|
||||
from PIL import ImageColor, Image, ImageDraw, ImageFont
|
||||
|
||||
import networks
|
||||
import tools
|
||||
|
||||
to_np = lambda x: x.detach().cpu().numpy()
|
||||
|
||||
|
||||
def symlog(x):
|
||||
return torch.sign(x) * torch.log(torch.abs(x) + 1.0)
|
||||
|
||||
|
||||
def symexp(x):
|
||||
return torch.sign(x) * (torch.exp(torch.abs(x)) - 1.0)
|
||||
|
||||
|
||||
class RewardEMA(object):
|
||||
"""running mean and std"""
|
||||
|
||||
def __init__(self, device, alpha=1e-2):
|
||||
self.device = device
|
||||
self.scale = torch.zeros((1,)).to(device)
|
||||
self.alpha = alpha
|
||||
self.range = torch.tensor([0.05, 0.95]).to(device)
|
||||
|
||||
def __call__(self, x):
|
||||
flat_x = torch.flatten(x.detach())
|
||||
x_quantile = torch.quantile(input=flat_x, q=self.range)
|
||||
scale = x_quantile[1] - x_quantile[0]
|
||||
new_scale = self.alpha * scale + (1 - self.alpha) * self.scale
|
||||
self.scale = new_scale
|
||||
return x / torch.clip(self.scale, min=1.0)
|
||||
|
||||
|
||||
class WorldModel(nn.Module):
|
||||
def __init__(self, step, config):
|
||||
super(WorldModel, self).__init__()
|
||||
self._step = step
|
||||
self._use_amp = True if config.precision == 16 else False
|
||||
self._config = config
|
||||
self.encoder = networks.ConvEncoder(
|
||||
config.grayscale,
|
||||
config.cnn_depth,
|
||||
config.act,
|
||||
config.norm,
|
||||
config.encoder_kernels,
|
||||
)
|
||||
if config.size[0] == 64 and config.size[1] == 64:
|
||||
embed_size = (
|
||||
(64 // 2 ** (len(config.encoder_kernels))) ** 2
|
||||
* config.cnn_depth
|
||||
* 2 ** (len(config.encoder_kernels) - 1)
|
||||
)
|
||||
else:
|
||||
raise NotImplemented(f"{config.size} is not applicable now")
|
||||
self.dynamics = networks.RSSM(
|
||||
config.dyn_stoch,
|
||||
config.dyn_deter,
|
||||
config.dyn_hidden,
|
||||
config.dyn_input_layers,
|
||||
config.dyn_output_layers,
|
||||
config.dyn_rec_depth,
|
||||
config.dyn_shared,
|
||||
config.dyn_discrete,
|
||||
config.act,
|
||||
config.norm,
|
||||
config.dyn_mean_act,
|
||||
config.dyn_std_act,
|
||||
config.dyn_temp_post,
|
||||
config.dyn_min_std,
|
||||
config.dyn_cell,
|
||||
config.unimix_ratio,
|
||||
config.num_actions,
|
||||
embed_size,
|
||||
config.device,
|
||||
)
|
||||
self.heads = nn.ModuleDict()
|
||||
channels = 1 if config.grayscale else 3
|
||||
shape = (channels,) + config.size
|
||||
if config.dyn_discrete:
|
||||
feat_size = config.dyn_stoch * config.dyn_discrete + config.dyn_deter
|
||||
else:
|
||||
feat_size = config.dyn_stoch + config.dyn_deter
|
||||
self.heads["image"] = networks.ConvDecoder(
|
||||
feat_size, # pytorch version
|
||||
config.cnn_depth,
|
||||
config.act,
|
||||
config.norm,
|
||||
shape,
|
||||
config.decoder_kernels,
|
||||
)
|
||||
if config.reward_head == "twohot":
|
||||
self.heads["reward"] = networks.DenseHead(
|
||||
feat_size, # pytorch version
|
||||
(255,),
|
||||
config.reward_layers,
|
||||
config.units,
|
||||
config.act,
|
||||
config.norm,
|
||||
dist=config.reward_head,
|
||||
)
|
||||
else:
|
||||
self.heads["reward"] = networks.DenseHead(
|
||||
feat_size, # pytorch version
|
||||
[],
|
||||
config.reward_layers,
|
||||
config.units,
|
||||
config.act,
|
||||
config.norm,
|
||||
dist=config.reward_head,
|
||||
)
|
||||
# added this
|
||||
self.heads["reward"].apply(tools.weight_init)
|
||||
if config.pred_discount:
|
||||
self.heads["discount"] = networks.DenseHead(
|
||||
feat_size, # pytorch version
|
||||
[],
|
||||
config.discount_layers,
|
||||
config.units,
|
||||
config.act,
|
||||
config.norm,
|
||||
dist="binary",
|
||||
)
|
||||
for name in config.grad_heads:
|
||||
assert name in self.heads, name
|
||||
self._model_opt = tools.Optimizer(
|
||||
"model",
|
||||
self.parameters(),
|
||||
config.model_lr,
|
||||
config.opt_eps,
|
||||
config.grad_clip,
|
||||
config.weight_decay,
|
||||
opt=config.opt,
|
||||
use_amp=self._use_amp,
|
||||
)
|
||||
self._scales = dict(reward=config.reward_scale, discount=config.discount_scale)
|
||||
|
||||
def _train(self, data):
|
||||
# action (batch_size, batch_length, act_dim)
|
||||
# image (batch_size, batch_length, h, w, ch)
|
||||
# reward (batch_size, batch_length)
|
||||
# discount (batch_size, batch_length)
|
||||
data = self.preprocess(data)
|
||||
|
||||
with tools.RequiresGrad(self):
|
||||
with torch.cuda.amp.autocast(self._use_amp):
|
||||
embed = self.encoder(data)
|
||||
post, prior = self.dynamics.observe(embed, data["action"])
|
||||
kl_free = tools.schedule(self._config.kl_free, self._step)
|
||||
kl_lscale = tools.schedule(self._config.kl_lscale, self._step)
|
||||
kl_rscale = tools.schedule(self._config.kl_rscale, self._step)
|
||||
kl_loss, kl_value, loss_lhs, loss_rhs = self.dynamics.kl_loss(
|
||||
post, prior, self._config.kl_forward, kl_free, kl_lscale, kl_rscale
|
||||
)
|
||||
losses = {}
|
||||
likes = {}
|
||||
for name, head in self.heads.items():
|
||||
grad_head = name in self._config.grad_heads
|
||||
feat = self.dynamics.get_feat(post)
|
||||
feat = feat if grad_head else feat.detach()
|
||||
pred = head(feat)
|
||||
# if name == 'image':
|
||||
# losses[name] = torch.nn.functional.mse_loss(pred.mode(), data[name], 'sum')
|
||||
like = pred.log_prob(data[name])
|
||||
likes[name] = like
|
||||
losses[name] = -torch.mean(like) * self._scales.get(name, 1.0)
|
||||
model_loss = sum(losses.values()) + kl_loss
|
||||
metrics = self._model_opt(model_loss, self.parameters())
|
||||
|
||||
metrics.update({f"{name}_loss": to_np(loss) for name, loss in losses.items()})
|
||||
metrics["kl_free"] = kl_free
|
||||
metrics["kl_lscale"] = kl_lscale
|
||||
metrics["kl_rscale"] = kl_rscale
|
||||
metrics["loss_lhs"] = to_np(loss_lhs)
|
||||
metrics["loss_rhs"] = to_np(loss_rhs)
|
||||
metrics["kl"] = to_np(torch.mean(kl_value))
|
||||
with torch.cuda.amp.autocast(self._use_amp):
|
||||
metrics["prior_ent"] = to_np(
|
||||
torch.mean(self.dynamics.get_dist(prior).entropy())
|
||||
)
|
||||
metrics["post_ent"] = to_np(
|
||||
torch.mean(self.dynamics.get_dist(post).entropy())
|
||||
)
|
||||
context = dict(
|
||||
embed=embed,
|
||||
feat=self.dynamics.get_feat(post),
|
||||
kl=kl_value,
|
||||
postent=self.dynamics.get_dist(post).entropy(),
|
||||
)
|
||||
post = {k: v.detach() for k, v in post.items()}
|
||||
return post, context, metrics
|
||||
|
||||
def preprocess(self, obs):
|
||||
obs = obs.copy()
|
||||
if self._config.obs_trans == "normalize":
|
||||
obs["image"] = torch.Tensor(obs["image"]) / 255.0 - 0.5
|
||||
elif self._config.obs_trans == "identity":
|
||||
obs["image"] = torch.Tensor(obs["image"])
|
||||
elif self._config.obs_trans == "symlog":
|
||||
obs["image"] = symlog(torch.Tensor(obs["image"]))
|
||||
else:
|
||||
raise NotImplemented(f"{self._config.reward_trans} is not implemented")
|
||||
if self._config.reward_trans == "tanh":
|
||||
# (batch_size, batch_length) -> (batch_size, batch_length, 1)
|
||||
obs["reward"] = torch.tanh(torch.Tensor(obs["reward"])).unsqueeze(-1)
|
||||
elif self._config.reward_trans == "identity":
|
||||
# (batch_size, batch_length) -> (batch_size, batch_length, 1)
|
||||
obs["reward"] = torch.Tensor(obs["reward"]).unsqueeze(-1)
|
||||
elif self._config.reward_trans == "symlog":
|
||||
obs["reward"] = symlog(torch.Tensor(obs["reward"])).unsqueeze(-1)
|
||||
else:
|
||||
raise NotImplemented(f"{self._config.reward_trans} is not implemented")
|
||||
if "discount" in obs:
|
||||
obs["discount"] *= self._config.discount
|
||||
# (batch_size, batch_length) -> (batch_size, batch_length, 1)
|
||||
obs["discount"] = torch.Tensor(obs["discount"]).unsqueeze(-1)
|
||||
obs = {k: torch.Tensor(v).to(self._config.device) for k, v in obs.items()}
|
||||
return obs
|
||||
|
||||
def video_pred(self, data):
|
||||
data = self.preprocess(data)
|
||||
embed = self.encoder(data)
|
||||
|
||||
states, _ = self.dynamics.observe(embed[:6, :5], data["action"][:6, :5])
|
||||
recon = self.heads["image"](self.dynamics.get_feat(states)).mode()[:6]
|
||||
reward_post = self.heads["reward"](self.dynamics.get_feat(states)).mode()[:6]
|
||||
init = {k: v[:, -1] for k, v in states.items()}
|
||||
prior = self.dynamics.imagine(data["action"][:6, 5:], init)
|
||||
openl = self.heads["image"](self.dynamics.get_feat(prior)).mode()
|
||||
reward_prior = self.heads["reward"](self.dynamics.get_feat(prior)).mode()
|
||||
# observed image is given until 5 steps
|
||||
model = torch.cat([recon[:, :5], openl], 1)
|
||||
if self._config.obs_trans == "normalize":
|
||||
truth = data["image"][:6] + 0.5
|
||||
model += 0.5
|
||||
elif self._config.obs_trans == "symlog":
|
||||
truth = symexp(data["image"][:6]) / 255.0
|
||||
model = symexp(model) / 255.0
|
||||
error = (model - truth + 1) / 2
|
||||
|
||||
return torch.cat([truth, model, error], 2)
|
||||
|
||||
|
||||
class ImagBehavior(nn.Module):
|
||||
def __init__(self, config, world_model, stop_grad_actor=True, reward=None):
|
||||
super(ImagBehavior, self).__init__()
|
||||
self._use_amp = True if config.precision == 16 else False
|
||||
self._config = config
|
||||
self._world_model = world_model
|
||||
self._stop_grad_actor = stop_grad_actor
|
||||
self._reward = reward
|
||||
if config.dyn_discrete:
|
||||
feat_size = config.dyn_stoch * config.dyn_discrete + config.dyn_deter
|
||||
else:
|
||||
feat_size = config.dyn_stoch + config.dyn_deter
|
||||
self.actor = networks.ActionHead(
|
||||
feat_size, # pytorch version
|
||||
config.num_actions,
|
||||
config.actor_layers,
|
||||
config.units,
|
||||
config.act,
|
||||
config.norm,
|
||||
config.actor_dist,
|
||||
config.actor_init_std,
|
||||
config.actor_min_std,
|
||||
config.actor_dist,
|
||||
config.actor_temp,
|
||||
config.actor_outscale,
|
||||
) # action_dist -> action_disc?
|
||||
if config.value_head == "twohot":
|
||||
self.value = networks.DenseHead(
|
||||
feat_size, # pytorch version
|
||||
(255,),
|
||||
config.value_layers,
|
||||
config.units,
|
||||
config.act,
|
||||
config.norm,
|
||||
config.value_head,
|
||||
)
|
||||
else:
|
||||
self.value = networks.DenseHead(
|
||||
feat_size, # pytorch version
|
||||
[],
|
||||
config.value_layers,
|
||||
config.units,
|
||||
config.act,
|
||||
config.norm,
|
||||
config.value_head,
|
||||
)
|
||||
self.value.apply(tools.weight_init)
|
||||
if config.slow_value_target or config.slow_actor_target:
|
||||
self._slow_value = copy.deepcopy(self.value)
|
||||
self._updates = 0
|
||||
kw = dict(wd=config.weight_decay, opt=config.opt, use_amp=self._use_amp)
|
||||
self._actor_opt = tools.Optimizer(
|
||||
"actor",
|
||||
self.actor.parameters(),
|
||||
config.actor_lr,
|
||||
config.ac_opt_eps,
|
||||
config.actor_grad_clip,
|
||||
**kw,
|
||||
)
|
||||
self._value_opt = tools.Optimizer(
|
||||
"value",
|
||||
self.value.parameters(),
|
||||
config.value_lr,
|
||||
config.ac_opt_eps,
|
||||
config.value_grad_clip,
|
||||
**kw,
|
||||
)
|
||||
if self._config.reward_EMA:
|
||||
self.reward_ema = RewardEMA(device=self._config.device)
|
||||
|
||||
def _train(
|
||||
self,
|
||||
start,
|
||||
objective=None,
|
||||
action=None,
|
||||
reward=None,
|
||||
imagine=None,
|
||||
tape=None,
|
||||
repeats=None,
|
||||
):
|
||||
objective = objective or self._reward
|
||||
self._update_slow_target()
|
||||
metrics = {}
|
||||
|
||||
with tools.RequiresGrad(self.actor):
|
||||
with torch.cuda.amp.autocast(self._use_amp):
|
||||
imag_feat, imag_state, imag_action = self._imagine(
|
||||
start, self.actor, self._config.imag_horizon, repeats
|
||||
)
|
||||
reward = objective(imag_feat, imag_state, imag_action)
|
||||
if self._config.reward_trans == "symlog":
|
||||
# rescale predicted reward by head['reward']
|
||||
reward = symexp(reward)
|
||||
actor_ent = self.actor(imag_feat).entropy()
|
||||
state_ent = self._world_model.dynamics.get_dist(imag_state).entropy()
|
||||
# this target is not scaled
|
||||
# slow is flag to indicate whether slow_target is used for lambda-return
|
||||
target, weights = self._compute_target(
|
||||
imag_feat,
|
||||
imag_state,
|
||||
imag_action,
|
||||
reward,
|
||||
actor_ent,
|
||||
state_ent,
|
||||
self._config.slow_actor_target,
|
||||
)
|
||||
actor_loss, mets = self._compute_actor_loss(
|
||||
imag_feat,
|
||||
imag_state,
|
||||
imag_action,
|
||||
target,
|
||||
actor_ent,
|
||||
state_ent,
|
||||
weights,
|
||||
)
|
||||
metrics.update(mets)
|
||||
if self._config.slow_value_target != self._config.slow_actor_target:
|
||||
target, weights = self._compute_target(
|
||||
imag_feat,
|
||||
imag_state,
|
||||
imag_action,
|
||||
reward,
|
||||
actor_ent,
|
||||
state_ent,
|
||||
self._config.slow_value_target,
|
||||
)
|
||||
value_input = imag_feat
|
||||
|
||||
with tools.RequiresGrad(self.value):
|
||||
with torch.cuda.amp.autocast(self._use_amp):
|
||||
value = self.value(value_input[:-1].detach())
|
||||
target = torch.stack(target, dim=1)
|
||||
# only critic target is processed using symlog(not actor)
|
||||
if self._config.critic_trans == "symlog":
|
||||
metrics["unscaled_target_mean"] = to_np(torch.mean(target))
|
||||
target = symlog(target)
|
||||
# (time, batch, 1), (time, batch, 1) -> (time, batch)
|
||||
value_loss = -value.log_prob(target.detach())
|
||||
if self._config.value_decay:
|
||||
value_loss += self._config.value_decay * value.mode()
|
||||
# (time, batch, 1), (time, batch, 1) -> (1,)
|
||||
value_loss = torch.mean(weights[:-1] * value_loss[:, :, None])
|
||||
|
||||
metrics["value_mean"] = to_np(torch.mean(value.mode()))
|
||||
metrics["value_max"] = to_np(torch.max(value.mode()))
|
||||
metrics["value_min"] = to_np(torch.min(value.mode()))
|
||||
metrics["value_std"] = to_np(torch.std(value.mode()))
|
||||
metrics["target_mean"] = to_np(torch.mean(target))
|
||||
metrics["reward_mean"] = to_np(torch.mean(reward))
|
||||
metrics["reward_std"] = to_np(torch.std(reward))
|
||||
metrics["actor_ent"] = to_np(torch.mean(actor_ent))
|
||||
with tools.RequiresGrad(self):
|
||||
metrics.update(self._actor_opt(actor_loss, self.actor.parameters()))
|
||||
metrics.update(self._value_opt(value_loss, self.value.parameters()))
|
||||
return imag_feat, imag_state, imag_action, weights, metrics
|
||||
|
||||
def _imagine(self, start, policy, horizon, repeats=None):
|
||||
dynamics = self._world_model.dynamics
|
||||
if repeats:
|
||||
raise NotImplemented("repeats is not implemented in this version")
|
||||
flatten = lambda x: x.reshape([-1] + list(x.shape[2:]))
|
||||
start = {k: flatten(v) for k, v in start.items()}
|
||||
|
||||
def step(prev, _):
|
||||
state, _, _ = prev
|
||||
feat = dynamics.get_feat(state)
|
||||
inp = feat.detach() if self._stop_grad_actor else feat
|
||||
action = policy(inp).sample()
|
||||
succ = dynamics.img_step(state, action, sample=self._config.imag_sample)
|
||||
return succ, feat, action
|
||||
|
||||
feat = 0 * dynamics.get_feat(start)
|
||||
action = policy(feat).mode()
|
||||
succ, feats, actions = tools.static_scan(
|
||||
step, [torch.arange(horizon)], (start, feat, action)
|
||||
)
|
||||
states = {k: torch.cat([start[k][None], v[:-1]], 0) for k, v in succ.items()}
|
||||
if repeats:
|
||||
raise NotImplemented("repeats is not implemented in this version")
|
||||
|
||||
return feats, states, actions
|
||||
|
||||
def _compute_target(
|
||||
self, imag_feat, imag_state, imag_action, reward, actor_ent, state_ent, slow
|
||||
):
|
||||
if "discount" in self._world_model.heads:
|
||||
inp = self._world_model.dynamics.get_feat(imag_state)
|
||||
discount = self._world_model.heads["discount"](inp).mean
|
||||
else:
|
||||
discount = self._config.discount * torch.ones_like(reward)
|
||||
if self._config.future_entropy and self._config.actor_entropy() > 0:
|
||||
reward += self._config.actor_entropy() * actor_ent
|
||||
if self._config.future_entropy and self._config.actor_state_entropy() > 0:
|
||||
reward += self._config.actor_state_entropy() * state_ent
|
||||
if slow:
|
||||
value = self._slow_value(imag_feat).mode()
|
||||
else:
|
||||
value = self.value(imag_feat).mode()
|
||||
if self._config.critic_trans == "symlog":
|
||||
# After adding this line there is issue
|
||||
value = symexp(value)
|
||||
target = tools.lambda_return(
|
||||
reward[:-1],
|
||||
value[:-1],
|
||||
discount[:-1],
|
||||
bootstrap=value[-1],
|
||||
lambda_=self._config.discount_lambda,
|
||||
axis=0,
|
||||
)
|
||||
weights = torch.cumprod(
|
||||
torch.cat([torch.ones_like(discount[:1]), discount[:-1]], 0), 0
|
||||
).detach()
|
||||
return target, weights
|
||||
|
||||
def _compute_actor_loss(
|
||||
self, imag_feat, imag_state, imag_action, target, actor_ent, state_ent, weights
|
||||
):
|
||||
metrics = {}
|
||||
inp = imag_feat.detach() if self._stop_grad_actor else imag_feat
|
||||
policy = self.actor(inp)
|
||||
actor_ent = policy.entropy()
|
||||
# Q-val for actor is not transformed using symlog
|
||||
target = torch.stack(target, dim=1)
|
||||
if self._config.reward_EMA:
|
||||
target = self.reward_ema(target)
|
||||
metrics["EMA_scale"] = to_np(self.reward_ema.scale)
|
||||
|
||||
if self._config.imag_gradient == "dynamics":
|
||||
actor_target = target
|
||||
elif self._config.imag_gradient == "reinforce":
|
||||
actor_target = (
|
||||
policy.log_prob(imag_action)[:-1][:, :, None]
|
||||
* (target - self.value(imag_feat[:-1]).mode()).detach()
|
||||
)
|
||||
elif self._config.imag_gradient == "both":
|
||||
actor_target = (
|
||||
policy.log_prob(imag_action)[:-1][:, :, None]
|
||||
* (target - self.value(imag_feat[:-1]).mode()).detach()
|
||||
)
|
||||
mix = self._config.imag_gradient_mix()
|
||||
actor_target = mix * target + (1 - mix) * actor_target
|
||||
metrics["imag_gradient_mix"] = mix
|
||||
else:
|
||||
raise NotImplementedError(self._config.imag_gradient)
|
||||
if not self._config.future_entropy and (self._config.actor_entropy() > 0):
|
||||
actor_entropy = self._config.actor_entropy() * actor_ent[:-1][:, :, None]
|
||||
actor_target += actor_entropy
|
||||
metrics["actor_entropy"] = to_np(torch.mean(actor_entropy))
|
||||
if not self._config.future_entropy and (self._config.actor_state_entropy() > 0):
|
||||
state_entropy = self._config.actor_state_entropy() * state_ent[:-1]
|
||||
actor_target += state_entropy
|
||||
metrics["actor_state_entropy"] = to_np(torch.mean(state_entropy))
|
||||
actor_loss = -torch.mean(weights[:-1] * actor_target)
|
||||
return actor_loss, metrics
|
||||
|
||||
def _update_slow_target(self):
|
||||
if self._config.slow_value_target or self._config.slow_actor_target:
|
||||
if self._updates % self._config.slow_target_update == 0:
|
||||
mix = self._config.slow_target_fraction
|
||||
for s, d in zip(self.value.parameters(), self._slow_value.parameters()):
|
||||
d.data = mix * s.data + (1 - mix) * d.data
|
||||
self._updates += 1
|
631
networks.py
Normal file
631
networks.py
Normal file
@ -0,0 +1,631 @@
|
||||
import math
|
||||
import numpy as np
|
||||
|
||||
import torch
|
||||
from torch import nn
|
||||
import torch.nn.functional as F
|
||||
from torch import distributions as torchd
|
||||
|
||||
import tools
|
||||
|
||||
|
||||
class RSSM(nn.Module):
|
||||
def __init__(
|
||||
self,
|
||||
stoch=30,
|
||||
deter=200,
|
||||
hidden=200,
|
||||
layers_input=1,
|
||||
layers_output=1,
|
||||
rec_depth=1,
|
||||
shared=False,
|
||||
discrete=False,
|
||||
act=nn.ELU,
|
||||
norm=nn.LayerNorm,
|
||||
mean_act="none",
|
||||
std_act="softplus",
|
||||
temp_post=True,
|
||||
min_std=0.1,
|
||||
cell="gru",
|
||||
unimix_ratio=0.01,
|
||||
num_actions=None,
|
||||
embed=None,
|
||||
device=None,
|
||||
):
|
||||
super(RSSM, self).__init__()
|
||||
self._stoch = stoch
|
||||
self._deter = deter
|
||||
self._hidden = hidden
|
||||
self._min_std = min_std
|
||||
self._layers_input = layers_input
|
||||
self._layers_output = layers_output
|
||||
self._rec_depth = rec_depth
|
||||
self._shared = shared
|
||||
self._discrete = discrete
|
||||
self._act = act
|
||||
self._norm = norm
|
||||
self._mean_act = mean_act
|
||||
self._std_act = std_act
|
||||
self._temp_post = temp_post
|
||||
self._unimix_ratio = unimix_ratio
|
||||
self._embed = embed
|
||||
self._device = device
|
||||
|
||||
inp_layers = []
|
||||
if self._discrete:
|
||||
inp_dim = self._stoch * self._discrete + num_actions
|
||||
else:
|
||||
inp_dim = self._stoch + num_actions
|
||||
if self._shared:
|
||||
inp_dim += self._embed
|
||||
for i in range(self._layers_input):
|
||||
inp_layers.append(nn.Linear(inp_dim, self._hidden))
|
||||
inp_layers.append(self._act())
|
||||
if i == 0:
|
||||
inp_dim = self._hidden
|
||||
self._inp_layers = nn.Sequential(*inp_layers)
|
||||
|
||||
if cell == "gru":
|
||||
self._cell = GRUCell(self._hidden, self._deter)
|
||||
elif cell == "gru_layer_norm":
|
||||
self._cell = GRUCell(self._hidden, self._deter, norm=True)
|
||||
else:
|
||||
raise NotImplementedError(cell)
|
||||
|
||||
img_out_layers = []
|
||||
inp_dim = self._deter
|
||||
for i in range(self._layers_output):
|
||||
img_out_layers.append(nn.Linear(inp_dim, self._hidden))
|
||||
img_out_layers.append(self._norm(self._hidden))
|
||||
img_out_layers.append(self._act())
|
||||
if i == 0:
|
||||
inp_dim = self._hidden
|
||||
self._img_out_layers = nn.Sequential(*img_out_layers)
|
||||
|
||||
obs_out_layers = []
|
||||
if self._temp_post:
|
||||
inp_dim = self._deter + self._embed
|
||||
else:
|
||||
inp_dim = self._embed
|
||||
for i in range(self._layers_output):
|
||||
obs_out_layers.append(nn.Linear(inp_dim, self._hidden))
|
||||
obs_out_layers.append(self._norm(self._hidden))
|
||||
obs_out_layers.append(self._act())
|
||||
if i == 0:
|
||||
inp_dim = self._hidden
|
||||
self._obs_out_layers = nn.Sequential(*obs_out_layers)
|
||||
|
||||
if self._discrete:
|
||||
self._ims_stat_layer = nn.Linear(self._hidden, self._stoch * self._discrete)
|
||||
self._obs_stat_layer = nn.Linear(self._hidden, self._stoch * self._discrete)
|
||||
else:
|
||||
self._ims_stat_layer = nn.Linear(self._hidden, 2 * self._stoch)
|
||||
self._obs_stat_layer = nn.Linear(self._hidden, 2 * self._stoch)
|
||||
|
||||
def initial(self, batch_size):
|
||||
deter = torch.zeros(batch_size, self._deter).to(self._device)
|
||||
if self._discrete:
|
||||
state = dict(
|
||||
logit=torch.zeros([batch_size, self._stoch, self._discrete]).to(
|
||||
self._device
|
||||
),
|
||||
stoch=torch.zeros([batch_size, self._stoch, self._discrete]).to(
|
||||
self._device
|
||||
),
|
||||
deter=deter,
|
||||
)
|
||||
else:
|
||||
state = dict(
|
||||
mean=torch.zeros([batch_size, self._stoch]).to(self._device),
|
||||
std=torch.zeros([batch_size, self._stoch]).to(self._device),
|
||||
stoch=torch.zeros([batch_size, self._stoch]).to(self._device),
|
||||
deter=deter,
|
||||
)
|
||||
return state
|
||||
|
||||
def observe(self, embed, action, state=None):
|
||||
swap = lambda x: x.permute([1, 0] + list(range(2, len(x.shape))))
|
||||
if state is None:
|
||||
state = self.initial(action.shape[0])
|
||||
# (batch, time, ch) -> (time, batch, ch)
|
||||
embed, action = swap(embed), swap(action)
|
||||
post, prior = tools.static_scan(
|
||||
lambda prev_state, prev_act, embed: self.obs_step(
|
||||
prev_state[0], prev_act, embed
|
||||
),
|
||||
(action, embed),
|
||||
(state, state),
|
||||
)
|
||||
|
||||
# (batch, time, stoch, discrete_num) -> (batch, time, stoch, discrete_num)
|
||||
post = {k: swap(v) for k, v in post.items()}
|
||||
prior = {k: swap(v) for k, v in prior.items()}
|
||||
return post, prior
|
||||
|
||||
def imagine(self, action, state=None):
|
||||
swap = lambda x: x.permute([1, 0] + list(range(2, len(x.shape))))
|
||||
if state is None:
|
||||
state = self.initial(action.shape[0])
|
||||
assert isinstance(state, dict), state
|
||||
action = action
|
||||
action = swap(action)
|
||||
prior = tools.static_scan(self.img_step, [action], state)
|
||||
prior = prior[0]
|
||||
prior = {k: swap(v) for k, v in prior.items()}
|
||||
return prior
|
||||
|
||||
def get_feat(self, state):
|
||||
stoch = state["stoch"]
|
||||
if self._discrete:
|
||||
shape = list(stoch.shape[:-2]) + [self._stoch * self._discrete]
|
||||
stoch = stoch.reshape(shape)
|
||||
return torch.cat([stoch, state["deter"]], -1)
|
||||
|
||||
def get_dist(self, state, dtype=None):
|
||||
if self._discrete:
|
||||
logit = state["logit"]
|
||||
dist = torchd.independent.Independent(
|
||||
tools.OneHotDist(logit, unimix_ratio=self._unimix_ratio), 1
|
||||
)
|
||||
else:
|
||||
mean, std = state["mean"], state["std"]
|
||||
dist = tools.ContDist(
|
||||
torchd.independent.Independent(torchd.normal.Normal(mean, std), 1)
|
||||
)
|
||||
return dist
|
||||
|
||||
def obs_step(self, prev_state, prev_action, embed, sample=True):
|
||||
# if shared is True, prior and post both use same networks(inp_layers, _img_out_layers, _ims_stat_layer)
|
||||
# otherwise, post use different network(_obs_out_layers) with prior[deter] and embed as inputs
|
||||
prior = self.img_step(prev_state, prev_action, None, sample)
|
||||
if self._shared:
|
||||
post = self.img_step(prev_state, prev_action, embed, sample)
|
||||
else:
|
||||
if self._temp_post:
|
||||
x = torch.cat([prior["deter"], embed], -1)
|
||||
else:
|
||||
x = embed
|
||||
# (batch_size, prior_deter + embed) -> (batch_size, hidden)
|
||||
x = self._obs_out_layers(x)
|
||||
# (batch_size, hidden) -> (batch_size, stoch, discrete_num)
|
||||
stats = self._suff_stats_layer("obs", x)
|
||||
if sample:
|
||||
stoch = self.get_dist(stats).sample()
|
||||
else:
|
||||
stoch = self.get_dist(stats).mode()
|
||||
post = {"stoch": stoch, "deter": prior["deter"], **stats}
|
||||
return post, prior
|
||||
|
||||
# this is used for making future image
|
||||
def img_step(self, prev_state, prev_action, embed=None, sample=True):
|
||||
# (batch, stoch, discrete_num)
|
||||
prev_stoch = prev_state["stoch"]
|
||||
if self._discrete:
|
||||
shape = list(prev_stoch.shape[:-2]) + [self._stoch * self._discrete]
|
||||
# (batch, stoch, discrete_num) -> (batch, stoch * discrete_num)
|
||||
prev_stoch = prev_stoch.reshape(shape)
|
||||
if self._shared:
|
||||
if embed is None:
|
||||
shape = list(prev_action.shape[:-1]) + [self._embed]
|
||||
embed = torch.zeros(shape)
|
||||
# (batch, stoch * discrete_num) -> (batch, stoch * discrete_num + action, embed)
|
||||
x = torch.cat([prev_stoch, prev_action, embed], -1)
|
||||
else:
|
||||
x = torch.cat([prev_stoch, prev_action], -1)
|
||||
# (batch, stoch * discrete_num + action, embed) -> (batch, hidden)
|
||||
x = self._inp_layers(x)
|
||||
for _ in range(self._rec_depth): # rec depth is not correctly implemented
|
||||
deter = prev_state["deter"]
|
||||
# (batch, hidden), (batch, deter) -> (batch, deter), (batch, deter)
|
||||
x, deter = self._cell(x, [deter])
|
||||
deter = deter[0] # Keras wraps the state in a list.
|
||||
# (batch, deter) -> (batch, hidden)
|
||||
x = self._img_out_layers(x)
|
||||
# (batch, hidden) -> (batch_size, stoch, discrete_num)
|
||||
stats = self._suff_stats_layer("ims", x)
|
||||
if sample:
|
||||
stoch = self.get_dist(stats).sample()
|
||||
else:
|
||||
stoch = self.get_dist(stats).mode()
|
||||
prior = {"stoch": stoch, "deter": deter, **stats}
|
||||
return prior
|
||||
|
||||
def _suff_stats_layer(self, name, x):
|
||||
if self._discrete:
|
||||
if name == "ims":
|
||||
x = self._ims_stat_layer(x)
|
||||
elif name == "obs":
|
||||
x = self._obs_stat_layer(x)
|
||||
else:
|
||||
raise NotImplementedError
|
||||
logit = x.reshape(list(x.shape[:-1]) + [self._stoch, self._discrete])
|
||||
return {"logit": logit}
|
||||
else:
|
||||
if name == "ims":
|
||||
x = self._ims_stat_layer(x)
|
||||
elif name == "obs":
|
||||
x = self._obs_stat_layer(x)
|
||||
else:
|
||||
raise NotImplementedError
|
||||
mean, std = torch.split(x, [self._stoch] * 2, -1)
|
||||
mean = {
|
||||
"none": lambda: mean,
|
||||
"tanh5": lambda: 5.0 * torch.tanh(mean / 5.0),
|
||||
}[self._mean_act]()
|
||||
std = {
|
||||
"softplus": lambda: torch.softplus(std),
|
||||
"abs": lambda: torch.abs(std + 1),
|
||||
"sigmoid": lambda: torch.sigmoid(std),
|
||||
"sigmoid2": lambda: 2 * torch.sigmoid(std / 2),
|
||||
}[self._std_act]()
|
||||
std = std + self._min_std
|
||||
return {"mean": mean, "std": std}
|
||||
|
||||
def kl_loss(self, post, prior, forward, free, lscale, rscale):
|
||||
kld = torchd.kl.kl_divergence
|
||||
dist = lambda x: self.get_dist(x)
|
||||
sg = lambda x: {k: v.detach() for k, v in x.items()}
|
||||
# forward == false -> (post, prior)
|
||||
lhs, rhs = (prior, post) if forward else (post, prior)
|
||||
|
||||
# forward == false -> Lrep
|
||||
value_lhs = value = kld(
|
||||
dist(lhs) if self._discrete else dist(lhs)._dist,
|
||||
dist(sg(rhs)) if self._discrete else dist(sg(rhs))._dist,
|
||||
)
|
||||
# forward == false -> Ldyn
|
||||
value_rhs = kld(
|
||||
dist(sg(lhs)) if self._discrete else dist(sg(lhs))._dist,
|
||||
dist(rhs) if self._discrete else dist(rhs)._dist,
|
||||
)
|
||||
loss_lhs = torch.clip(torch.mean(value_lhs), min=free)
|
||||
loss_rhs = torch.clip(torch.mean(value_rhs), min=free)
|
||||
loss = lscale * loss_lhs + rscale * loss_rhs
|
||||
|
||||
return loss, value, loss_lhs, loss_rhs
|
||||
|
||||
|
||||
class ConvEncoder(nn.Module):
|
||||
def __init__(
|
||||
self,
|
||||
grayscale=False,
|
||||
depth=32,
|
||||
act=nn.ELU,
|
||||
norm=nn.LayerNorm,
|
||||
kernels=(3, 3, 3, 3),
|
||||
):
|
||||
super(ConvEncoder, self).__init__()
|
||||
self._act = act
|
||||
self._norm = norm
|
||||
self._depth = depth
|
||||
self._kernels = kernels
|
||||
h, w = 64, 64
|
||||
layers = []
|
||||
for i, kernel in enumerate(self._kernels):
|
||||
if i == 0:
|
||||
if grayscale:
|
||||
inp_dim = 1
|
||||
else:
|
||||
inp_dim = 3
|
||||
else:
|
||||
inp_dim = 2 ** (i - 1) * self._depth
|
||||
depth = 2**i * self._depth
|
||||
layers.append(
|
||||
Conv2dSame(
|
||||
in_channels=inp_dim,
|
||||
out_channels=depth,
|
||||
kernel_size=(kernel, kernel),
|
||||
stride=(2, 2),
|
||||
)
|
||||
)
|
||||
h, w = h // 2, w // 2
|
||||
# layers.append(norm([depth, h, w]))
|
||||
layers.append(act())
|
||||
self.layers = nn.Sequential(*layers)
|
||||
|
||||
def __call__(self, obs):
|
||||
x = obs["image"].reshape((-1,) + tuple(obs["image"].shape[-3:]))
|
||||
x = x.permute(0, 3, 1, 2)
|
||||
x = self.layers(x)
|
||||
# prod: product of all elements
|
||||
x = x.reshape([x.shape[0], np.prod(x.shape[1:])])
|
||||
shape = list(obs["image"].shape[:-3]) + [x.shape[-1]]
|
||||
return x.reshape(shape)
|
||||
|
||||
|
||||
class ConvDecoder(nn.Module):
|
||||
def __init__(
|
||||
self,
|
||||
inp_depth,
|
||||
depth=32,
|
||||
act=nn.ELU,
|
||||
norm=nn.LayerNorm,
|
||||
shape=(3, 64, 64),
|
||||
kernels=(3, 3, 3, 3),
|
||||
):
|
||||
super(ConvDecoder, self).__init__()
|
||||
self._inp_depth = inp_depth
|
||||
self._act = act
|
||||
self._norm = norm
|
||||
self._depth = depth
|
||||
self._shape = shape
|
||||
self._kernels = kernels
|
||||
self._embed_size = (
|
||||
(64 // 2 ** (len(kernels))) ** 2 * depth * 2 ** (len(kernels) - 1)
|
||||
)
|
||||
|
||||
self._linear_layer = nn.Linear(inp_depth, self._embed_size)
|
||||
inp_dim = self._embed_size // 16
|
||||
|
||||
cnnt_layers = []
|
||||
h, w = 4, 4
|
||||
for i, kernel in enumerate(self._kernels):
|
||||
depth = self._embed_size // 16 // (2 ** (i + 1))
|
||||
act = self._act
|
||||
if i == len(self._kernels) - 1:
|
||||
depth = self._shape[0]
|
||||
act = None
|
||||
if i != 0:
|
||||
inp_dim = 2 ** (len(self._kernels) - (i - 1) - 2) * self._depth
|
||||
pad_h, outpad_h = calc_same_pad(k=kernel, s=2, d=1)
|
||||
pad_w, outpad_w = calc_same_pad(k=kernel, s=2, d=1)
|
||||
cnnt_layers.append(
|
||||
nn.ConvTranspose2d(
|
||||
inp_dim,
|
||||
depth,
|
||||
kernel,
|
||||
2,
|
||||
padding=(pad_h, pad_w),
|
||||
output_padding=(outpad_h, outpad_w),
|
||||
)
|
||||
)
|
||||
h, w = h * 2, w * 2
|
||||
# cnnt_layers.append(norm([depth, h, w]))
|
||||
if act is not None:
|
||||
cnnt_layers.append(act())
|
||||
self._cnnt_layers = nn.Sequential(*cnnt_layers)
|
||||
|
||||
def __call__(self, features, dtype=None):
|
||||
x = self._linear_layer(features)
|
||||
x = x.reshape([-1, 4, 4, self._embed_size // 16])
|
||||
x = x.permute(0, 3, 1, 2)
|
||||
x = self._cnnt_layers(x)
|
||||
mean = x.reshape(features.shape[:-1] + self._shape)
|
||||
mean = mean.permute(0, 1, 3, 4, 2)
|
||||
return tools.ContDist(
|
||||
torchd.independent.Independent(
|
||||
torchd.normal.Normal(mean, 1), len(self._shape)
|
||||
)
|
||||
)
|
||||
|
||||
|
||||
class DenseHead(nn.Module):
|
||||
def __init__(
|
||||
self,
|
||||
inp_dim,
|
||||
shape,
|
||||
layers,
|
||||
units,
|
||||
act=nn.ELU,
|
||||
norm=nn.LayerNorm,
|
||||
dist="normal",
|
||||
std=1.0,
|
||||
unimix_ratio=0.0,
|
||||
):
|
||||
super(DenseHead, self).__init__()
|
||||
self._shape = (shape,) if isinstance(shape, int) else shape
|
||||
if len(self._shape) == 0:
|
||||
self._shape = (1,)
|
||||
self._layers = layers
|
||||
self._units = units
|
||||
self._act = act
|
||||
self._norm = norm
|
||||
self._dist = dist
|
||||
self._std = std
|
||||
self._unimix_ratio = unimix_ratio
|
||||
|
||||
mean_layers = []
|
||||
for index in range(self._layers):
|
||||
mean_layers.append(nn.Linear(inp_dim, self._units))
|
||||
mean_layers.append(norm(self._units))
|
||||
mean_layers.append(act())
|
||||
if index == 0:
|
||||
inp_dim = self._units
|
||||
mean_layers.append(nn.Linear(inp_dim, np.prod(self._shape)))
|
||||
self._mean_layers = nn.Sequential(*mean_layers)
|
||||
|
||||
if self._std == "learned":
|
||||
self._std_layer = nn.Linear(self._units, np.prod(self._shape))
|
||||
|
||||
def __call__(self, features, dtype=None):
|
||||
x = features
|
||||
mean = self._mean_layers(x)
|
||||
if self._std == "learned":
|
||||
std = self._std_layer(x)
|
||||
std = torch.softplus(std) + 0.01
|
||||
else:
|
||||
std = self._std
|
||||
if self._dist == "normal":
|
||||
return tools.ContDist(
|
||||
torchd.independent.Independent(
|
||||
torchd.normal.Normal(mean, std), len(self._shape)
|
||||
)
|
||||
)
|
||||
if self._dist == "huber":
|
||||
return tools.ContDist(
|
||||
torchd.independent.Independent(
|
||||
tools.UnnormalizedHuber(mean, std, 1.0), len(self._shape)
|
||||
)
|
||||
)
|
||||
if self._dist == "binary":
|
||||
return tools.Bernoulli(
|
||||
torchd.independent.Independent(
|
||||
torchd.bernoulli.Bernoulli(logits=mean), len(self._shape)
|
||||
)
|
||||
)
|
||||
if self._dist == "twohot":
|
||||
return tools.TwoHotDist(logits=mean, unimix_ratio=self._unimix_ratio)
|
||||
raise NotImplementedError(self._dist)
|
||||
|
||||
|
||||
class ActionHead(nn.Module):
|
||||
def __init__(
|
||||
self,
|
||||
inp_dim,
|
||||
size,
|
||||
layers,
|
||||
units,
|
||||
act=nn.ELU,
|
||||
norm=nn.LayerNorm,
|
||||
dist="trunc_normal",
|
||||
init_std=0.0,
|
||||
min_std=0.1,
|
||||
action_disc=5,
|
||||
temp=0.1,
|
||||
outscale=0,
|
||||
):
|
||||
super(ActionHead, self).__init__()
|
||||
self._size = size
|
||||
self._layers = layers
|
||||
self._units = units
|
||||
self._dist = dist
|
||||
self._act = act
|
||||
self._norm = norm
|
||||
self._min_std = min_std
|
||||
self._init_std = init_std
|
||||
self._action_disc = action_disc
|
||||
self._temp = temp() if callable(temp) else temp
|
||||
self._outscale = outscale
|
||||
|
||||
pre_layers = []
|
||||
for index in range(self._layers):
|
||||
pre_layers.append(nn.Linear(inp_dim, self._units))
|
||||
pre_layers.append(norm(self._units))
|
||||
pre_layers.append(act())
|
||||
if index == 0:
|
||||
inp_dim = self._units
|
||||
self._pre_layers = nn.Sequential(*pre_layers)
|
||||
|
||||
if self._dist in ["tanh_normal", "tanh_normal_5", "normal", "trunc_normal"]:
|
||||
self._dist_layer = nn.Linear(self._units, 2 * self._size)
|
||||
elif self._dist in ["normal_1", "onehot", "onehot_gumbel"]:
|
||||
self._dist_layer = nn.Linear(self._units, self._size)
|
||||
|
||||
def __call__(self, features, dtype=None):
|
||||
x = features
|
||||
x = self._pre_layers(x)
|
||||
if self._dist == "tanh_normal":
|
||||
x = self._dist_layer(x)
|
||||
mean, std = torch.split(x, 2, -1)
|
||||
mean = torch.tanh(mean)
|
||||
std = F.softplus(std + self._init_std) + self._min_std
|
||||
dist = torchd.normal.Normal(mean, std)
|
||||
dist = torchd.transformed_distribution.TransformedDistribution(
|
||||
dist, tools.TanhBijector()
|
||||
)
|
||||
dist = torchd.independent.Independent(dist, 1)
|
||||
dist = tools.SampleDist(dist)
|
||||
elif self._dist == "tanh_normal_5":
|
||||
x = self._dist_layer(x)
|
||||
mean, std = torch.split(x, 2, -1)
|
||||
mean = 5 * torch.tanh(mean / 5)
|
||||
std = F.softplus(std + 5) + 5
|
||||
dist = torchd.normal.Normal(mean, std)
|
||||
dist = torchd.transformed_distribution.TransformedDistribution(
|
||||
dist, tools.TanhBijector()
|
||||
)
|
||||
dist = torchd.independent.Independent(dist, 1)
|
||||
dist = tools.SampleDist(dist)
|
||||
elif self._dist == "normal":
|
||||
x = self._dist_layer(x)
|
||||
mean, std = torch.split(x, 2, -1)
|
||||
std = F.softplus(std + self._init_std) + self._min_std
|
||||
dist = torchd.normal.Normal(mean, std)
|
||||
dist = tools.ContDist(torchd.independent.Independent(dist, 1))
|
||||
elif self._dist == "normal_1":
|
||||
x = self._dist_layer(x)
|
||||
dist = torchd.normal.Normal(mean, 1)
|
||||
dist = tools.ContDist(torchd.independent.Independent(dist, 1))
|
||||
elif self._dist == "trunc_normal":
|
||||
x = self._dist_layer(x)
|
||||
mean, std = torch.split(x, [self._size] * 2, -1)
|
||||
mean = torch.tanh(mean)
|
||||
std = 2 * torch.sigmoid(std / 2) + self._min_std
|
||||
dist = tools.SafeTruncatedNormal(mean, std, -1, 1)
|
||||
dist = tools.ContDist(torchd.independent.Independent(dist, 1))
|
||||
elif self._dist == "onehot":
|
||||
x = self._dist_layer(x)
|
||||
dist = tools.OneHotDist(x)
|
||||
elif self._dist == "onehot_gumble":
|
||||
x = self._dist_layer(x)
|
||||
temp = self._temp
|
||||
dist = tools.ContDist(torchd.gumbel.Gumbel(x, 1 / temp))
|
||||
else:
|
||||
raise NotImplementedError(self._dist)
|
||||
return dist
|
||||
|
||||
|
||||
class GRUCell(nn.Module):
|
||||
def __init__(self, inp_size, size, norm=False, act=torch.tanh, update_bias=-1):
|
||||
super(GRUCell, self).__init__()
|
||||
self._inp_size = inp_size
|
||||
self._size = size
|
||||
self._act = act
|
||||
self._norm = norm
|
||||
self._update_bias = update_bias
|
||||
self._layer = nn.Linear(inp_size + size, 3 * size, bias=norm is not None)
|
||||
if norm:
|
||||
self._norm = nn.LayerNorm(3 * size)
|
||||
|
||||
@property
|
||||
def state_size(self):
|
||||
return self._size
|
||||
|
||||
def forward(self, inputs, state):
|
||||
state = state[0] # Keras wraps the state in a list.
|
||||
parts = self._layer(torch.cat([inputs, state], -1))
|
||||
if self._norm:
|
||||
parts = self._norm(parts)
|
||||
reset, cand, update = torch.split(parts, [self._size] * 3, -1)
|
||||
reset = torch.sigmoid(reset)
|
||||
cand = self._act(reset * cand)
|
||||
update = torch.sigmoid(update + self._update_bias)
|
||||
output = update * cand + (1 - update) * state
|
||||
return output, [output]
|
||||
|
||||
|
||||
class Conv2dSame(torch.nn.Conv2d):
|
||||
def calc_same_pad(self, i, k, s, d):
|
||||
return max((math.ceil(i / s) - 1) * s + (k - 1) * d + 1 - i, 0)
|
||||
|
||||
def forward(self, x):
|
||||
ih, iw = x.size()[-2:]
|
||||
pad_h = self.calc_same_pad(
|
||||
i=ih, k=self.kernel_size[0], s=self.stride[0], d=self.dilation[0]
|
||||
)
|
||||
pad_w = self.calc_same_pad(
|
||||
i=iw, k=self.kernel_size[1], s=self.stride[1], d=self.dilation[1]
|
||||
)
|
||||
|
||||
if pad_h > 0 or pad_w > 0:
|
||||
x = F.pad(
|
||||
x, [pad_w // 2, pad_w - pad_w // 2, pad_h // 2, pad_h - pad_h // 2]
|
||||
)
|
||||
|
||||
ret = F.conv2d(
|
||||
x,
|
||||
self.weight,
|
||||
self.bias,
|
||||
self.stride,
|
||||
self.padding,
|
||||
self.dilation,
|
||||
self.groups,
|
||||
)
|
||||
return ret
|
||||
|
||||
|
||||
def calc_same_pad(k, s, d):
|
||||
val = d * (k - 1) - s + 1
|
||||
pad = math.ceil(val / 2)
|
||||
outpad = pad * 2 - val
|
||||
return pad, outpad
|
12
requirements.txt
Normal file
12
requirements.txt
Normal file
@ -0,0 +1,12 @@
|
||||
torch==1.13.0
|
||||
numpy==1.20.1
|
||||
torchvision==0.14.0
|
||||
tensorboard==2.5.0
|
||||
pandas==1.2.4
|
||||
matplotlib==3.4.1
|
||||
ruamel.yaml==0.17.4
|
||||
gym[atari]==0.18.0
|
||||
moviepy==1.0.3
|
||||
einops==0.3.0
|
||||
protobuf==3.20.0
|
||||
dm_control==1.0.9
|
700
tools.py
Normal file
700
tools.py
Normal file
@ -0,0 +1,700 @@
|
||||
import datetime
|
||||
import io
|
||||
import json
|
||||
import pathlib
|
||||
import pickle
|
||||
import re
|
||||
import time
|
||||
import uuid
|
||||
|
||||
import numpy as np
|
||||
|
||||
import torch
|
||||
from torch import nn
|
||||
from torch.nn import functional as F
|
||||
from torch import distributions as torchd
|
||||
from torch.utils.data import Dataset
|
||||
from torch.utils.tensorboard import SummaryWriter
|
||||
|
||||
|
||||
class RequiresGrad:
|
||||
|
||||
def __init__(self, model):
|
||||
self._model = model
|
||||
|
||||
def __enter__(self):
|
||||
self._model.requires_grad_(requires_grad=True)
|
||||
|
||||
def __exit__(self, *args):
|
||||
self._model.requires_grad_(requires_grad=False)
|
||||
|
||||
|
||||
class TimeRecording:
|
||||
|
||||
def __init__(self, comment):
|
||||
self._comment = comment
|
||||
|
||||
def __enter__(self):
|
||||
self._st = torch.cuda.Event(enable_timing=True)
|
||||
self._nd = torch.cuda.Event(enable_timing=True)
|
||||
self._st.record()
|
||||
|
||||
def __exit__(self, *args):
|
||||
self._nd.record()
|
||||
torch.cuda.synchronize()
|
||||
print(self._comment, self._st.elapsed_time(self._nd)/1000)
|
||||
|
||||
|
||||
class Logger:
|
||||
|
||||
def __init__(self, logdir, step):
|
||||
self._logdir = logdir
|
||||
self._writer = SummaryWriter(log_dir=str(logdir), max_queue=1000)
|
||||
self._last_step = None
|
||||
self._last_time = None
|
||||
self._scalars = {}
|
||||
self._images = {}
|
||||
self._videos = {}
|
||||
self.step = step
|
||||
|
||||
def scalar(self, name, value):
|
||||
self._scalars[name] = float(value)
|
||||
|
||||
def image(self, name, value):
|
||||
self._images[name] = np.array(value)
|
||||
|
||||
def video(self, name, value):
|
||||
self._videos[name] = np.array(value)
|
||||
|
||||
def write(self, fps=False):
|
||||
scalars = list(self._scalars.items())
|
||||
if fps:
|
||||
scalars.append(('fps', self._compute_fps(self.step)))
|
||||
print(f'[{self.step}]', ' / '.join(f'{k} {v:.1f}' for k, v in scalars))
|
||||
with (self._logdir / 'metrics.jsonl').open('a') as f:
|
||||
f.write(json.dumps({'step': self.step, ** dict(scalars)}) + '\n')
|
||||
for name, value in scalars:
|
||||
self._writer.add_scalar('scalars/' + name, value, self.step)
|
||||
for name, value in self._images.items():
|
||||
self._writer.add_image(name, value, self.step)
|
||||
for name, value in self._videos.items():
|
||||
name = name if isinstance(name, str) else name.decode('utf-8')
|
||||
if np.issubdtype(value.dtype, np.floating):
|
||||
value = np.clip(255 * value, 0, 255).astype(np.uint8)
|
||||
B, T, H, W, C = value.shape
|
||||
value = value.transpose(1, 4, 2, 0, 3).reshape((1, T, C, H, B*W))
|
||||
self._writer.add_video(name, value, self.step, 16)
|
||||
|
||||
self._writer.flush()
|
||||
self._scalars = {}
|
||||
self._images = {}
|
||||
self._videos = {}
|
||||
|
||||
def _compute_fps(self, step):
|
||||
if self._last_step is None:
|
||||
self._last_time = time.time()
|
||||
self._last_step = step
|
||||
return 0
|
||||
steps = step - self._last_step
|
||||
duration = time.time() - self._last_time
|
||||
self._last_time += duration
|
||||
self._last_step = step
|
||||
return steps / duration
|
||||
|
||||
def offline_scalar(self, name, value, step):
|
||||
self._writer.add_scalar('scalars/'+name, value, step)
|
||||
|
||||
def offline_video(self, name, value, step):
|
||||
if np.issubdtype(value.dtype, np.floating):
|
||||
value = np.clip(255 * value, 0, 255).astype(np.uint8)
|
||||
B, T, H, W, C = value.shape
|
||||
value = value.transpose(1, 4, 2, 0, 3).reshape((1, T, C, H, B*W))
|
||||
self._writer.add_video(name, value, step, 16)
|
||||
|
||||
|
||||
def simulate(agent, envs, steps=0, episodes=0, state=None):
|
||||
# Initialize or unpack simulation state.
|
||||
if state is None:
|
||||
step, episode = 0, 0
|
||||
done = np.ones(len(envs), np.bool)
|
||||
length = np.zeros(len(envs), np.int32)
|
||||
obs = [None] * len(envs)
|
||||
agent_state = None
|
||||
reward = [0]*len(envs)
|
||||
else:
|
||||
step, episode, done, length, obs, agent_state, reward = state
|
||||
while (steps and step < steps) or (episodes and episode < episodes):
|
||||
# Reset envs if necessary.
|
||||
if done.any():
|
||||
indices = [index for index, d in enumerate(done) if d]
|
||||
results = [envs[i].reset() for i in indices]
|
||||
for index, result in zip(indices, results):
|
||||
obs[index] = result
|
||||
reward = [reward[i]*(1-done[i]) for i in range(len(envs))]
|
||||
# Step agents.
|
||||
obs = {k: np.stack([o[k] for o in obs]) for k in obs[0]}
|
||||
action, agent_state = agent(obs, done, agent_state, reward)
|
||||
if isinstance(action, dict):
|
||||
action = [
|
||||
{k: np.array(action[k][i].detach().cpu()) for k in action}
|
||||
for i in range(len(envs))]
|
||||
else:
|
||||
action = np.array(action)
|
||||
assert len(action) == len(envs)
|
||||
# Step envs.
|
||||
results = [e.step(a) for e, a in zip(envs, action)]
|
||||
obs, reward, done = zip(*[p[:3] for p in results])
|
||||
obs = list(obs)
|
||||
reward = list(reward)
|
||||
done = np.stack(done)
|
||||
episode += int(done.sum())
|
||||
length += 1
|
||||
step += (done * length).sum()
|
||||
length *= (1 - done)
|
||||
|
||||
return (step - steps, episode - episodes, done, length, obs, agent_state, reward)
|
||||
|
||||
|
||||
def save_episodes(directory, episodes):
|
||||
directory = pathlib.Path(directory).expanduser()
|
||||
directory.mkdir(parents=True, exist_ok=True)
|
||||
timestamp = datetime.datetime.now().strftime('%Y%m%dT%H%M%S')
|
||||
filenames = []
|
||||
for episode in episodes:
|
||||
identifier = str(uuid.uuid4().hex)
|
||||
length = len(episode['reward'])
|
||||
filename = directory / f'{timestamp}-{identifier}-{length}.npz'
|
||||
with io.BytesIO() as f1:
|
||||
np.savez_compressed(f1, **episode)
|
||||
f1.seek(0)
|
||||
with filename.open('wb') as f2:
|
||||
f2.write(f1.read())
|
||||
filenames.append(filename)
|
||||
return filenames
|
||||
|
||||
|
||||
def from_generator(generator, batch_size):
|
||||
while True:
|
||||
batch = []
|
||||
for _ in range(batch_size):
|
||||
batch.append(next(generator))
|
||||
data = {}
|
||||
for key in batch[0].keys():
|
||||
data[key] = []
|
||||
for i in range(batch_size):
|
||||
data[key].append(batch[i][key])
|
||||
data[key] = np.stack(data[key], 0)
|
||||
yield data
|
||||
|
||||
|
||||
def sample_episodes(episodes, length=None, balance=False, seed=0):
|
||||
random = np.random.RandomState(seed)
|
||||
while True:
|
||||
episode = random.choice(list(episodes.values()))
|
||||
if length:
|
||||
total = len(next(iter(episode.values())))
|
||||
available = total - length
|
||||
if available < 1:
|
||||
print(f'Skipped short episode of length {available}.')
|
||||
continue
|
||||
if balance:
|
||||
index = min(random.randint(0, total), available)
|
||||
else:
|
||||
index = int(random.randint(0, available + 1))
|
||||
episode = {k: v[index: index + length] for k, v in episode.items()}
|
||||
yield episode
|
||||
|
||||
|
||||
def load_episodes(directory, limit=None, reverse=True):
|
||||
directory = pathlib.Path(directory).expanduser()
|
||||
episodes = {}
|
||||
total = 0
|
||||
if reverse:
|
||||
for filename in reversed(sorted(directory.glob('*.npz'))):
|
||||
try:
|
||||
with filename.open('rb') as f:
|
||||
episode = np.load(f)
|
||||
episode = {k: episode[k] for k in episode.keys()}
|
||||
except Exception as e:
|
||||
print(f'Could not load episode: {e}')
|
||||
continue
|
||||
episodes[str(filename)] = episode
|
||||
total += len(episode['reward']) - 1
|
||||
if limit and total >= limit:
|
||||
break
|
||||
else:
|
||||
for filename in sorted(directory.glob('*.npz')):
|
||||
try:
|
||||
with filename.open('rb') as f:
|
||||
episode = np.load(f)
|
||||
episode = {k: episode[k] for k in episode.keys()}
|
||||
except Exception as e:
|
||||
print(f'Could not load episode: {e}')
|
||||
continue
|
||||
episodes[str(filename)] = episode
|
||||
total += len(episode['reward']) - 1
|
||||
if limit and total >= limit:
|
||||
break
|
||||
return episodes
|
||||
|
||||
|
||||
class SampleDist:
|
||||
|
||||
def __init__(self, dist, samples=100):
|
||||
self._dist = dist
|
||||
self._samples = samples
|
||||
|
||||
@property
|
||||
def name(self):
|
||||
return 'SampleDist'
|
||||
|
||||
def __getattr__(self, name):
|
||||
return getattr(self._dist, name)
|
||||
|
||||
def mean(self):
|
||||
samples = self._dist.sample(self._samples)
|
||||
return torch.mean(samples, 0)
|
||||
|
||||
def mode(self):
|
||||
sample = self._dist.sample(self._samples)
|
||||
logprob = self._dist.log_prob(sample)
|
||||
return sample[torch.argmax(logprob)][0]
|
||||
|
||||
def entropy(self):
|
||||
sample = self._dist.sample(self._samples)
|
||||
logprob = self.log_prob(sample)
|
||||
return -torch.mean(logprob, 0)
|
||||
|
||||
|
||||
class OneHotDist(torchd.one_hot_categorical.OneHotCategorical):
|
||||
|
||||
def __init__(self, logits=None, probs=None, unimix_ratio=0.0):
|
||||
if logits is not None and probs is None and unimix_ratio > 0.0:
|
||||
probs = F.softmax(logits, dim=-1)
|
||||
probs = probs * (1.0-unimix_ratio) + unimix_ratio / probs.shape[-1]
|
||||
logits = None
|
||||
super().__init__(logits=logits, probs=probs)
|
||||
|
||||
def mode(self):
|
||||
_mode = F.one_hot(torch.argmax(super().logits, axis=-1), super().logits.shape[-1])
|
||||
return _mode.detach() + super().logits - super().logits.detach()
|
||||
|
||||
def sample(self, sample_shape=(), seed=None):
|
||||
if seed is not None:
|
||||
raise ValueError('need to check')
|
||||
sample = super().sample(sample_shape)
|
||||
probs = super().probs
|
||||
while len(probs.shape) < len(sample.shape):
|
||||
probs = probs[None]
|
||||
sample += probs - probs.detach()
|
||||
return sample
|
||||
|
||||
|
||||
class TwoHotDist(torchd.one_hot_categorical.OneHotCategorical):
|
||||
|
||||
def __init__(self, logits=None, probs=None, unimix_ratio=0.0, device='cuda'):
|
||||
if logits is not None and probs is None and unimix_ratio > 0.0:
|
||||
probs = F.softmax(logits, dim=-1)
|
||||
probs = probs * (1.0-unimix_ratio) + unimix_ratio / probs.shape[-1]
|
||||
logits = None
|
||||
super().__init__(logits=logits, probs=probs)
|
||||
|
||||
self.buckets = torch.linspace(-20.0, 20.0, steps=255).to(device)
|
||||
self.width = (self.buckets[-1] - self.buckets[0]) / 255
|
||||
|
||||
def mode(self):
|
||||
_mode = super().probs * self.buckets
|
||||
return torch.sum(_mode, dim=-1, keepdim=True)
|
||||
|
||||
# Inside OneHotCategorical, log_prob is calculated using only max element in targets
|
||||
def log_prob(self, x):
|
||||
# x(time, batch, 1)
|
||||
x = (x - self.buckets[0]) / self.width
|
||||
lower_indices = (x).to(torch.int64)
|
||||
# lower_indices is idnside 0 ~ len(buckets)-2
|
||||
lower_indices = torch.clip(lower_indices, max=len(self.buckets)-2)
|
||||
# upper_indices is inside 1 ~ len(buckets)-1
|
||||
upper_indices = lower_indices + 1
|
||||
lower_weight = torch.abs(x - upper_indices).squeeze(-1)
|
||||
upper_weight = torch.abs(x - lower_indices).squeeze(-1)
|
||||
# (time, batch, 1) -> (time, batch, bucket_class)
|
||||
lower_log_prob = super().log_prob(F.one_hot(lower_indices.squeeze(-1), num_classes=len(self.buckets)))
|
||||
upper_log_prob = super().log_prob(F.one_hot(upper_indices.squeeze(-1), num_classes=len(self.buckets)))
|
||||
|
||||
# label = lower_log_prob * lower_weight + upper_log_prob * upper_weight
|
||||
# # (time, batch, bucket_class) -> (time, batch)
|
||||
# cross_entropy = torch.sum(torch.log(super().probs) * label, axis=-1)
|
||||
|
||||
return lower_weight * lower_log_prob + upper_weight * upper_log_prob
|
||||
|
||||
class ContDist:
|
||||
|
||||
def __init__(self, dist=None):
|
||||
super().__init__()
|
||||
self._dist = dist
|
||||
self.mean = dist.mean
|
||||
|
||||
def __getattr__(self, name):
|
||||
return getattr(self._dist, name)
|
||||
|
||||
def entropy(self):
|
||||
return self._dist.entropy()
|
||||
|
||||
def mode(self):
|
||||
return self._dist.mean
|
||||
|
||||
def sample(self, sample_shape=()):
|
||||
return self._dist.rsample(sample_shape)
|
||||
|
||||
def log_prob(self, x):
|
||||
return self._dist.log_prob(x)
|
||||
|
||||
|
||||
class Bernoulli:
|
||||
|
||||
def __init__(self, dist=None):
|
||||
super().__init__()
|
||||
self._dist = dist
|
||||
self.mean = dist.mean
|
||||
|
||||
def __getattr__(self, name):
|
||||
return getattr(self._dist, name)
|
||||
|
||||
def entropy(self):
|
||||
return self._dist.entropy()
|
||||
|
||||
def mode(self):
|
||||
_mode = torch.round(self._dist.mean)
|
||||
return _mode.detach() +self._dist.mean - self._dist.mean.detach()
|
||||
|
||||
def sample(self, sample_shape=()):
|
||||
return self._dist.rsample(sample_shape)
|
||||
|
||||
def log_prob(self, x):
|
||||
_logits = self._dist.base_dist.logits
|
||||
log_probs0 = -F.softplus(_logits)
|
||||
log_probs1 = -F.softplus(-_logits)
|
||||
|
||||
return log_probs0 * (1-x) + log_probs1 * x
|
||||
|
||||
|
||||
class UnnormalizedHuber(torchd.normal.Normal):
|
||||
|
||||
def __init__(self, loc, scale, threshold=1, **kwargs):
|
||||
super().__init__(loc, scale, **kwargs)
|
||||
self._threshold = threshold
|
||||
|
||||
def log_prob(self, event):
|
||||
return -(torch.sqrt(
|
||||
(event - self.mean) ** 2 + self._threshold ** 2) - self._threshold)
|
||||
|
||||
def mode(self):
|
||||
return self.mean
|
||||
|
||||
|
||||
class SafeTruncatedNormal(torchd.normal.Normal):
|
||||
|
||||
def __init__(self, loc, scale, low, high, clip=1e-6, mult=1):
|
||||
super().__init__(loc, scale)
|
||||
self._low = low
|
||||
self._high = high
|
||||
self._clip = clip
|
||||
self._mult = mult
|
||||
|
||||
def sample(self, sample_shape):
|
||||
event = super().sample(sample_shape)
|
||||
if self._clip:
|
||||
clipped = torch.clip(event, self._low + self._clip,
|
||||
self._high - self._clip)
|
||||
event = event - event.detach() + clipped.detach()
|
||||
if self._mult:
|
||||
event *= self._mult
|
||||
return event
|
||||
|
||||
|
||||
class TanhBijector(torchd.Transform):
|
||||
|
||||
def __init__(self, validate_args=False, name='tanh'):
|
||||
super().__init__()
|
||||
|
||||
def _forward(self, x):
|
||||
return torch.tanh(x)
|
||||
|
||||
def _inverse(self, y):
|
||||
y = torch.where(
|
||||
(torch.abs(y) <= 1.),
|
||||
torch.clamp(y, -0.99999997, 0.99999997), y)
|
||||
y = torch.atanh(y)
|
||||
return y
|
||||
|
||||
def _forward_log_det_jacobian(self, x):
|
||||
log2 = torch.math.log(2.0)
|
||||
return 2.0 * (log2 - x - torch.softplus(-2.0 * x))
|
||||
|
||||
|
||||
def static_scan_for_lambda_return(fn, inputs, start):
|
||||
last = start
|
||||
indices = range(inputs[0].shape[0])
|
||||
indices = reversed(indices)
|
||||
flag = True
|
||||
for index in indices:
|
||||
inp = lambda x: (_input[x] for _input in inputs)
|
||||
last = fn(last, *inp(index))
|
||||
if flag:
|
||||
outputs = last
|
||||
flag = False
|
||||
else:
|
||||
outputs = torch.cat([outputs, last], dim=-1)
|
||||
outputs = torch.reshape(outputs, [outputs.shape[0], outputs.shape[1], 1])
|
||||
outputs = torch.unbind(outputs, dim=0)
|
||||
return outputs
|
||||
|
||||
|
||||
def lambda_return(
|
||||
reward, value, pcont, bootstrap, lambda_, axis):
|
||||
# Setting lambda=1 gives a discounted Monte Carlo return.
|
||||
# Setting lambda=0 gives a fixed 1-step return.
|
||||
#assert reward.shape.ndims == value.shape.ndims, (reward.shape, value.shape)
|
||||
assert len(reward.shape) == len(value.shape), (reward.shape, value.shape)
|
||||
if isinstance(pcont, (int, float)):
|
||||
pcont = pcont * torch.ones_like(reward)
|
||||
dims = list(range(len(reward.shape)))
|
||||
dims = [axis] + dims[1:axis] + [0] + dims[axis + 1:]
|
||||
if axis != 0:
|
||||
reward = reward.permute(dims)
|
||||
value = value.permute(dims)
|
||||
pcont = pcont.permute(dims)
|
||||
if bootstrap is None:
|
||||
bootstrap = torch.zeros_like(value[-1])
|
||||
next_values = torch.cat([value[1:], bootstrap[None]], 0)
|
||||
inputs = reward + pcont * next_values * (1 - lambda_)
|
||||
#returns = static_scan(
|
||||
# lambda agg, cur0, cur1: cur0 + cur1 * lambda_ * agg,
|
||||
# (inputs, pcont), bootstrap, reverse=True)
|
||||
# reimplement to optimize performance
|
||||
returns = static_scan_for_lambda_return(
|
||||
lambda agg, cur0, cur1: cur0 + cur1 * lambda_ * agg,
|
||||
(inputs, pcont), bootstrap)
|
||||
if axis != 0:
|
||||
returns = returns.permute(dims)
|
||||
return returns
|
||||
|
||||
|
||||
class Optimizer():
|
||||
|
||||
def __init__(
|
||||
self, name, parameters, lr, eps=1e-4, clip=None, wd=None, wd_pattern=r'.*',
|
||||
opt='adam', use_amp=False):
|
||||
assert 0 <= wd < 1
|
||||
assert not clip or 1 <= clip
|
||||
self._name = name
|
||||
self._parameters = parameters
|
||||
self._clip = clip
|
||||
self._wd = wd
|
||||
self._wd_pattern = wd_pattern
|
||||
self._opt = {
|
||||
'adam': lambda: torch.optim.Adam(parameters,
|
||||
lr=lr,
|
||||
eps=eps),
|
||||
'nadam': lambda: NotImplemented(
|
||||
f'{config.opt} is not implemented'),
|
||||
'adamax': lambda: torch.optim.Adamax(parameters,
|
||||
lr=lr,
|
||||
eps=eps),
|
||||
'sgd': lambda: torch.optim.SGD(parameters,
|
||||
lr=lr),
|
||||
'momentum': lambda: torch.optim.SGD(parameters,
|
||||
lr=lr,
|
||||
momentum=0.9),
|
||||
}[opt]()
|
||||
self._scaler = torch.cuda.amp.GradScaler(enabled=use_amp)
|
||||
|
||||
def __call__(self, loss, params, retain_graph=False):
|
||||
assert len(loss.shape) == 0, loss.shape
|
||||
metrics = {}
|
||||
metrics[f'{self._name}_loss'] = loss.detach().cpu().numpy()
|
||||
self._scaler.scale(loss).backward()
|
||||
self._scaler.unscale_(self._opt)
|
||||
#loss.backward(retain_graph=retain_graph)
|
||||
norm = torch.nn.utils.clip_grad_norm_(params, self._clip)
|
||||
if self._wd:
|
||||
self._apply_weight_decay(params)
|
||||
self._scaler.step(self._opt)
|
||||
self._scaler.update()
|
||||
#self._opt.step()
|
||||
self._opt.zero_grad()
|
||||
metrics[f'{self._name}_grad_norm'] = norm.item()
|
||||
return metrics
|
||||
|
||||
def _apply_weight_decay(self, varibs):
|
||||
nontrivial = (self._wd_pattern != r'.*')
|
||||
if nontrivial:
|
||||
raise NotImplementedError
|
||||
for var in varibs:
|
||||
var.data = (1 - self._wd) * var.data
|
||||
|
||||
|
||||
def args_type(default):
|
||||
def parse_string(x):
|
||||
if default is None:
|
||||
return x
|
||||
if isinstance(default, bool):
|
||||
return bool(['False', 'True'].index(x))
|
||||
if isinstance(default, int):
|
||||
return float(x) if ('e' in x or '.' in x) else int(x)
|
||||
if isinstance(default, (list, tuple)):
|
||||
return tuple(args_type(default[0])(y) for y in x.split(','))
|
||||
return type(default)(x)
|
||||
def parse_object(x):
|
||||
if isinstance(default, (list, tuple)):
|
||||
return tuple(x)
|
||||
return x
|
||||
return lambda x: parse_string(x) if isinstance(x, str) else parse_object(x)
|
||||
|
||||
|
||||
def static_scan(fn, inputs, start):
|
||||
last = start
|
||||
indices = range(inputs[0].shape[0])
|
||||
flag = True
|
||||
for index in indices:
|
||||
inp = lambda x: (_input[x] for _input in inputs)
|
||||
last = fn(last, *inp(index))
|
||||
if flag:
|
||||
if type(last) == type({}):
|
||||
outputs = {key: value.clone().unsqueeze(0) for key, value in last.items()}
|
||||
else:
|
||||
outputs = []
|
||||
for _last in last:
|
||||
if type(_last) == type({}):
|
||||
outputs.append({key: value.clone().unsqueeze(0) for key, value in _last.items()})
|
||||
else:
|
||||
outputs.append(_last.clone().unsqueeze(0))
|
||||
flag = False
|
||||
else:
|
||||
if type(last) == type({}):
|
||||
for key in last.keys():
|
||||
outputs[key] = torch.cat([outputs[key], last[key].unsqueeze(0)], dim=0)
|
||||
else:
|
||||
for j in range(len(outputs)):
|
||||
if type(last[j]) == type({}):
|
||||
for key in last[j].keys():
|
||||
outputs[j][key] = torch.cat([outputs[j][key],
|
||||
last[j][key].unsqueeze(0)], dim=0)
|
||||
else:
|
||||
outputs[j] = torch.cat([outputs[j], last[j].unsqueeze(0)], dim=0)
|
||||
if type(last) == type({}):
|
||||
outputs = [outputs]
|
||||
return outputs
|
||||
|
||||
|
||||
# Original version
|
||||
#def static_scan2(fn, inputs, start, reverse=False):
|
||||
# last = start
|
||||
# outputs = [[] for _ in range(len([start] if type(start)==type({}) else start))]
|
||||
# indices = range(inputs[0].shape[0])
|
||||
# if reverse:
|
||||
# indices = reversed(indices)
|
||||
# for index in indices:
|
||||
# inp = lambda x: (_input[x] for _input in inputs)
|
||||
# last = fn(last, *inp(index))
|
||||
# [o.append(l) for o, l in zip(outputs, [last] if type(last)==type({}) else last)]
|
||||
# if reverse:
|
||||
# outputs = [list(reversed(x)) for x in outputs]
|
||||
# res = [[]] * len(outputs)
|
||||
# for i in range(len(outputs)):
|
||||
# if type(outputs[i][0]) == type({}):
|
||||
# _res = {}
|
||||
# for key in outputs[i][0].keys():
|
||||
# _res[key] = []
|
||||
# for j in range(len(outputs[i])):
|
||||
# _res[key].append(outputs[i][j][key])
|
||||
# #_res[key] = torch.stack(_res[key], 0)
|
||||
# _res[key] = faster_stack(_res[key], 0)
|
||||
# else:
|
||||
# _res = outputs[i]
|
||||
# #_res = torch.stack(_res, 0)
|
||||
# _res = faster_stack(_res, 0)
|
||||
# res[i] = _res
|
||||
# return res
|
||||
|
||||
|
||||
class Every:
|
||||
|
||||
def __init__(self, every):
|
||||
self._every = every
|
||||
self._last = None
|
||||
|
||||
def __call__(self, step):
|
||||
if not self._every:
|
||||
return False
|
||||
if self._last is None:
|
||||
self._last = step
|
||||
return True
|
||||
if step >= self._last + self._every:
|
||||
self._last += self._every
|
||||
return True
|
||||
return False
|
||||
|
||||
|
||||
class Once:
|
||||
|
||||
def __init__(self):
|
||||
self._once = True
|
||||
|
||||
def __call__(self):
|
||||
if self._once:
|
||||
self._once = False
|
||||
return True
|
||||
return False
|
||||
|
||||
|
||||
class Until:
|
||||
|
||||
def __init__(self, until):
|
||||
self._until = until
|
||||
|
||||
def __call__(self, step):
|
||||
if not self._until:
|
||||
return True
|
||||
return step < self._until
|
||||
|
||||
|
||||
def schedule(string, step):
|
||||
try:
|
||||
return float(string)
|
||||
except ValueError:
|
||||
match = re.match(r'linear\((.+),(.+),(.+)\)', string)
|
||||
if match:
|
||||
initial, final, duration = [float(group) for group in match.groups()]
|
||||
mix = torch.clip(torch.Tensor([step / duration]), 0, 1)[0]
|
||||
return (1 - mix) * initial + mix * final
|
||||
match = re.match(r'warmup\((.+),(.+)\)', string)
|
||||
if match:
|
||||
warmup, value = [float(group) for group in match.groups()]
|
||||
scale = torch.clip(step / warmup, 0, 1)
|
||||
return scale * value
|
||||
match = re.match(r'exp\((.+),(.+),(.+)\)', string)
|
||||
if match:
|
||||
initial, final, halflife = [float(group) for group in match.groups()]
|
||||
return (initial - final) * 0.5 ** (step / halflife) + final
|
||||
match = re.match(r'horizon\((.+),(.+),(.+)\)', string)
|
||||
if match:
|
||||
initial, final, duration = [float(group) for group in match.groups()]
|
||||
mix = torch.clip(step / duration, 0, 1)
|
||||
horizon = (1 - mix) * initial + mix * final
|
||||
return 1 - 1 / horizon
|
||||
raise NotImplementedError(string)
|
||||
|
||||
def weight_init(m):
|
||||
if isinstance(m, nn.Linear):
|
||||
nn.init.orthogonal_(m.weight.data)
|
||||
if hasattr(m.bias, 'data'):
|
||||
m.bias.data.fill_(0.0)
|
||||
elif isinstance(m, nn.Conv2d) or isinstance(m, nn.ConvTranspose2d):
|
||||
gain = nn.init.calculate_gain('relu')
|
||||
nn.init.orthogonal_(m.weight.data, gain)
|
||||
if hasattr(m.bias, 'data'):
|
||||
m.bias.data.fill_(0.0)
|
||||
elif isinstance(m, nn.LayerNorm):
|
||||
if hasattr(m.bias, 'data'):
|
||||
m.bias.data.fill_(0.0)
|
419
wrappers.py
Normal file
419
wrappers.py
Normal file
@ -0,0 +1,419 @@
|
||||
import threading
|
||||
|
||||
import gym
|
||||
import numpy as np
|
||||
|
||||
|
||||
class DeepMindLabyrinth(object):
|
||||
ACTION_SET_DEFAULT = (
|
||||
(0, 0, 0, 1, 0, 0, 0), # Forward
|
||||
(0, 0, 0, -1, 0, 0, 0), # Backward
|
||||
(0, 0, -1, 0, 0, 0, 0), # Strafe Left
|
||||
(0, 0, 1, 0, 0, 0, 0), # Strafe Right
|
||||
(-20, 0, 0, 0, 0, 0, 0), # Look Left
|
||||
(20, 0, 0, 0, 0, 0, 0), # Look Right
|
||||
(-20, 0, 0, 1, 0, 0, 0), # Look Left + Forward
|
||||
(20, 0, 0, 1, 0, 0, 0), # Look Right + Forward
|
||||
(0, 0, 0, 0, 1, 0, 0), # Fire
|
||||
)
|
||||
|
||||
ACTION_SET_MEDIUM = (
|
||||
(0, 0, 0, 1, 0, 0, 0), # Forward
|
||||
(0, 0, 0, -1, 0, 0, 0), # Backward
|
||||
(0, 0, -1, 0, 0, 0, 0), # Strafe Left
|
||||
(0, 0, 1, 0, 0, 0, 0), # Strafe Right
|
||||
(-20, 0, 0, 0, 0, 0, 0), # Look Left
|
||||
(20, 0, 0, 0, 0, 0, 0), # Look Right
|
||||
(0, 0, 0, 0, 0, 0, 0), # Idle.
|
||||
)
|
||||
|
||||
ACTION_SET_SMALL = (
|
||||
(0, 0, 0, 1, 0, 0, 0), # Forward
|
||||
(-20, 0, 0, 0, 0, 0, 0), # Look Left
|
||||
(20, 0, 0, 0, 0, 0, 0), # Look Right
|
||||
)
|
||||
|
||||
def __init__(
|
||||
self,
|
||||
level,
|
||||
mode,
|
||||
action_repeat=4,
|
||||
render_size=(64, 64),
|
||||
action_set=ACTION_SET_DEFAULT,
|
||||
level_cache=None,
|
||||
seed=None,
|
||||
runfiles_path=None,
|
||||
):
|
||||
assert mode in ("train", "test")
|
||||
import deepmind_lab
|
||||
|
||||
if runfiles_path:
|
||||
print("Setting DMLab runfiles path:", runfiles_path)
|
||||
deepmind_lab.set_runfiles_path(runfiles_path)
|
||||
self._config = {}
|
||||
self._config["width"] = render_size[0]
|
||||
self._config["height"] = render_size[1]
|
||||
self._config["logLevel"] = "WARN"
|
||||
if mode == "test":
|
||||
self._config["allowHoldOutLevels"] = "true"
|
||||
self._config["mixerSeed"] = 0x600D5EED
|
||||
self._action_repeat = action_repeat
|
||||
self._random = np.random.RandomState(seed)
|
||||
self._env = deepmind_lab.Lab(
|
||||
level="contributed/dmlab30/" + level,
|
||||
observations=["RGB_INTERLEAVED"],
|
||||
config={k: str(v) for k, v in self._config.items()},
|
||||
level_cache=level_cache,
|
||||
)
|
||||
self._action_set = action_set
|
||||
self._last_image = None
|
||||
self._done = True
|
||||
|
||||
@property
|
||||
def observation_space(self):
|
||||
shape = (self._config["height"], self._config["width"], 3)
|
||||
space = gym.spaces.Box(low=0, high=255, shape=shape, dtype=np.uint8)
|
||||
return gym.spaces.Dict({"image": space})
|
||||
|
||||
@property
|
||||
def action_space(self):
|
||||
return gym.spaces.Discrete(len(self._action_set))
|
||||
|
||||
def reset(self):
|
||||
self._done = False
|
||||
self._env.reset(seed=self._random.randint(0, 2**31 - 1))
|
||||
obs = self._get_obs()
|
||||
return obs
|
||||
|
||||
def step(self, action):
|
||||
raw_action = np.array(self._action_set[action], np.intc)
|
||||
reward = self._env.step(raw_action, num_steps=self._action_repeat)
|
||||
self._done = not self._env.is_running()
|
||||
obs = self._get_obs()
|
||||
return obs, reward, self._done, {}
|
||||
|
||||
def render(self, *args, **kwargs):
|
||||
if kwargs.get("mode", "rgb_array") != "rgb_array":
|
||||
raise ValueError("Only render mode 'rgb_array' is supported.")
|
||||
del args # Unused
|
||||
del kwargs # Unused
|
||||
return self._last_image
|
||||
|
||||
def close(self):
|
||||
self._env.close()
|
||||
|
||||
def _get_obs(self):
|
||||
if self._done:
|
||||
image = 0 * self._last_image
|
||||
else:
|
||||
image = self._env.observations()["RGB_INTERLEAVED"]
|
||||
self._last_image = image
|
||||
return {"image": image}
|
||||
|
||||
|
||||
class DeepMindControl:
|
||||
def __init__(self, name, action_repeat=1, size=(64, 64), camera=None):
|
||||
domain, task = name.split("_", 1)
|
||||
if domain == "cup": # Only domain with multiple words.
|
||||
domain = "ball_in_cup"
|
||||
if isinstance(domain, str):
|
||||
from dm_control import suite
|
||||
|
||||
self._env = suite.load(domain, task)
|
||||
else:
|
||||
assert task is None
|
||||
self._env = domain()
|
||||
self._action_repeat = action_repeat
|
||||
self._size = size
|
||||
if camera is None:
|
||||
camera = dict(quadruped=2).get(domain, 0)
|
||||
self._camera = camera
|
||||
|
||||
@property
|
||||
def observation_space(self):
|
||||
spaces = {}
|
||||
for key, value in self._env.observation_spec().items():
|
||||
spaces[key] = gym.spaces.Box(-np.inf, np.inf, value.shape, dtype=np.float32)
|
||||
spaces["image"] = gym.spaces.Box(0, 255, self._size + (3,), dtype=np.uint8)
|
||||
return gym.spaces.Dict(spaces)
|
||||
|
||||
@property
|
||||
def action_space(self):
|
||||
spec = self._env.action_spec()
|
||||
return gym.spaces.Box(spec.minimum, spec.maximum, dtype=np.float32)
|
||||
|
||||
def step(self, action):
|
||||
assert np.isfinite(action).all(), action
|
||||
reward = 0
|
||||
for _ in range(self._action_repeat):
|
||||
time_step = self._env.step(action)
|
||||
reward += time_step.reward or 0
|
||||
if time_step.last():
|
||||
break
|
||||
obs = dict(time_step.observation)
|
||||
obs["image"] = self.render()
|
||||
done = time_step.last()
|
||||
info = {"discount": np.array(time_step.discount, np.float32)}
|
||||
return obs, reward, done, info
|
||||
|
||||
def reset(self):
|
||||
time_step = self._env.reset()
|
||||
obs = dict(time_step.observation)
|
||||
obs["image"] = self.render()
|
||||
return obs
|
||||
|
||||
def render(self, *args, **kwargs):
|
||||
if kwargs.get("mode", "rgb_array") != "rgb_array":
|
||||
raise ValueError("Only render mode 'rgb_array' is supported.")
|
||||
return self._env.physics.render(*self._size, camera_id=self._camera)
|
||||
|
||||
|
||||
class Atari:
|
||||
LOCK = threading.Lock()
|
||||
|
||||
def __init__(
|
||||
self,
|
||||
name,
|
||||
action_repeat=4,
|
||||
size=(84, 84),
|
||||
grayscale=True,
|
||||
noops=30,
|
||||
life_done=False,
|
||||
sticky_actions=True,
|
||||
all_actions=False,
|
||||
):
|
||||
assert size[0] == size[1]
|
||||
import gym.wrappers
|
||||
import gym.envs.atari
|
||||
|
||||
if name == "james_bond":
|
||||
name = "jamesbond"
|
||||
with self.LOCK:
|
||||
env = gym.envs.atari.AtariEnv(
|
||||
game=name,
|
||||
obs_type="image",
|
||||
frameskip=1,
|
||||
repeat_action_probability=0.25 if sticky_actions else 0.0,
|
||||
full_action_space=all_actions,
|
||||
)
|
||||
# Avoid unnecessary rendering in inner env.
|
||||
env._get_obs = lambda: None
|
||||
# Tell wrapper that the inner env has no action repeat.
|
||||
env.spec = gym.envs.registration.EnvSpec("NoFrameskip-v0")
|
||||
env = gym.wrappers.AtariPreprocessing(
|
||||
env, noops, action_repeat, size[0], life_done, grayscale
|
||||
)
|
||||
self._env = env
|
||||
self._grayscale = grayscale
|
||||
|
||||
@property
|
||||
def observation_space(self):
|
||||
return gym.spaces.Dict(
|
||||
{
|
||||
"image": self._env.observation_space,
|
||||
"ram": gym.spaces.Box(0, 255, (128,), np.uint8),
|
||||
}
|
||||
)
|
||||
|
||||
@property
|
||||
def action_space(self):
|
||||
return self._env.action_space
|
||||
|
||||
def close(self):
|
||||
return self._env.close()
|
||||
|
||||
def reset(self):
|
||||
with self.LOCK:
|
||||
image = self._env.reset()
|
||||
if self._grayscale:
|
||||
image = image[..., None]
|
||||
obs = {"image": image, "ram": self._env.env._get_ram()}
|
||||
return obs
|
||||
|
||||
def step(self, action):
|
||||
image, reward, done, info = self._env.step(action)
|
||||
if self._grayscale:
|
||||
image = image[..., None]
|
||||
obs = {"image": image, "ram": self._env.env._get_ram()}
|
||||
return obs, reward, done, info
|
||||
|
||||
def render(self, mode):
|
||||
return self._env.render(mode)
|
||||
|
||||
|
||||
class CollectDataset:
|
||||
def __init__(self, env, callbacks=None, precision=32):
|
||||
self._env = env
|
||||
self._callbacks = callbacks or ()
|
||||
self._precision = precision
|
||||
self._episode = None
|
||||
|
||||
def __getattr__(self, name):
|
||||
return getattr(self._env, name)
|
||||
|
||||
def step(self, action):
|
||||
obs, reward, done, info = self._env.step(action)
|
||||
obs = {k: self._convert(v) for k, v in obs.items()}
|
||||
transition = obs.copy()
|
||||
if isinstance(action, dict):
|
||||
transition.update(action)
|
||||
else:
|
||||
transition["action"] = action
|
||||
transition["reward"] = reward
|
||||
transition["discount"] = info.get("discount", np.array(1 - float(done)))
|
||||
self._episode.append(transition)
|
||||
if done:
|
||||
for key, value in self._episode[1].items():
|
||||
if key not in self._episode[0]:
|
||||
self._episode[0][key] = 0 * value
|
||||
episode = {k: [t[k] for t in self._episode] for k in self._episode[0]}
|
||||
episode = {k: self._convert(v) for k, v in episode.items()}
|
||||
info["episode"] = episode
|
||||
for callback in self._callbacks:
|
||||
callback(episode)
|
||||
return obs, reward, done, info
|
||||
|
||||
def reset(self):
|
||||
obs = self._env.reset()
|
||||
transition = obs.copy()
|
||||
# Missing keys will be filled with a zeroed out version of the first
|
||||
# transition, because we do not know what action information the agent will
|
||||
# pass yet.
|
||||
transition["reward"] = 0.0
|
||||
transition["discount"] = 1.0
|
||||
self._episode = [transition]
|
||||
return obs
|
||||
|
||||
def _convert(self, value):
|
||||
value = np.array(value)
|
||||
if np.issubdtype(value.dtype, np.floating):
|
||||
dtype = {16: np.float16, 32: np.float32, 64: np.float64}[self._precision]
|
||||
elif np.issubdtype(value.dtype, np.signedinteger):
|
||||
dtype = {16: np.int16, 32: np.int32, 64: np.int64}[self._precision]
|
||||
elif np.issubdtype(value.dtype, np.uint8):
|
||||
dtype = np.uint8
|
||||
else:
|
||||
raise NotImplementedError(value.dtype)
|
||||
return value.astype(dtype)
|
||||
|
||||
|
||||
class TimeLimit:
|
||||
def __init__(self, env, duration):
|
||||
self._env = env
|
||||
self._duration = duration
|
||||
self._step = None
|
||||
|
||||
def __getattr__(self, name):
|
||||
return getattr(self._env, name)
|
||||
|
||||
def step(self, action):
|
||||
assert self._step is not None, "Must reset environment."
|
||||
obs, reward, done, info = self._env.step(action)
|
||||
self._step += 1
|
||||
if self._step >= self._duration:
|
||||
done = True
|
||||
if "discount" not in info:
|
||||
info["discount"] = np.array(1.0).astype(np.float32)
|
||||
self._step = None
|
||||
return obs, reward, done, info
|
||||
|
||||
def reset(self):
|
||||
self._step = 0
|
||||
return self._env.reset()
|
||||
|
||||
|
||||
class NormalizeActions:
|
||||
def __init__(self, env):
|
||||
self._env = env
|
||||
self._mask = np.logical_and(
|
||||
np.isfinite(env.action_space.low), np.isfinite(env.action_space.high)
|
||||
)
|
||||
self._low = np.where(self._mask, env.action_space.low, -1)
|
||||
self._high = np.where(self._mask, env.action_space.high, 1)
|
||||
|
||||
def __getattr__(self, name):
|
||||
return getattr(self._env, name)
|
||||
|
||||
@property
|
||||
def action_space(self):
|
||||
low = np.where(self._mask, -np.ones_like(self._low), self._low)
|
||||
high = np.where(self._mask, np.ones_like(self._low), self._high)
|
||||
return gym.spaces.Box(low, high, dtype=np.float32)
|
||||
|
||||
def step(self, action):
|
||||
original = (action + 1) / 2 * (self._high - self._low) + self._low
|
||||
original = np.where(self._mask, original, action)
|
||||
return self._env.step(original)
|
||||
|
||||
|
||||
class OneHotAction:
|
||||
def __init__(self, env):
|
||||
assert isinstance(env.action_space, gym.spaces.Discrete)
|
||||
self._env = env
|
||||
self._random = np.random.RandomState()
|
||||
|
||||
def __getattr__(self, name):
|
||||
return getattr(self._env, name)
|
||||
|
||||
@property
|
||||
def action_space(self):
|
||||
shape = (self._env.action_space.n,)
|
||||
space = gym.spaces.Box(low=0, high=1, shape=shape, dtype=np.float32)
|
||||
space.sample = self._sample_action
|
||||
space.discrete = True
|
||||
return space
|
||||
|
||||
def step(self, action):
|
||||
index = np.argmax(action).astype(int)
|
||||
reference = np.zeros_like(action)
|
||||
reference[index] = 1
|
||||
if not np.allclose(reference, action):
|
||||
raise ValueError(f"Invalid one-hot action:\n{action}")
|
||||
return self._env.step(index)
|
||||
|
||||
def reset(self):
|
||||
return self._env.reset()
|
||||
|
||||
def _sample_action(self):
|
||||
actions = self._env.action_space.n
|
||||
index = self._random.randint(0, actions)
|
||||
reference = np.zeros(actions, dtype=np.float32)
|
||||
reference[index] = 1.0
|
||||
return reference
|
||||
|
||||
|
||||
class RewardObs:
|
||||
def __init__(self, env):
|
||||
self._env = env
|
||||
|
||||
def __getattr__(self, name):
|
||||
return getattr(self._env, name)
|
||||
|
||||
@property
|
||||
def observation_space(self):
|
||||
spaces = self._env.observation_space.spaces
|
||||
assert "reward" not in spaces
|
||||
spaces["reward"] = gym.spaces.Box(-np.inf, np.inf, dtype=np.float32)
|
||||
return gym.spaces.Dict(spaces)
|
||||
|
||||
def step(self, action):
|
||||
obs, reward, done, info = self._env.step(action)
|
||||
obs["reward"] = reward
|
||||
return obs, reward, done, info
|
||||
|
||||
def reset(self):
|
||||
obs = self._env.reset()
|
||||
obs["reward"] = 0.0
|
||||
return obs
|
||||
|
||||
|
||||
class SelectAction:
|
||||
def __init__(self, env, key):
|
||||
self._env = env
|
||||
self._key = key
|
||||
|
||||
def __getattr__(self, name):
|
||||
return getattr(self._env, name)
|
||||
|
||||
def step(self, action):
|
||||
return self._env.step(action[self._key])
|
Loading…
x
Reference in New Issue
Block a user