Closes#952
- `SamplingConfig` supports `batch_size=None`. #1077
- tests and examples are covered by `mypy`. #1077
- `NetBase` is more used, stricter typing by making it generic. #1077
- `utils.net.common.Recurrent` now receives and returns a
`RecurrentStateBatch` instead of a dict. #1077
---------
Co-authored-by: Michael Panchenko <m.panchenko@appliedai.de>
Closes: #1058
### Api Extensions
- Batch received two new methods: `to_dict` and `to_list_of_dicts`.
#1063
- `Collector`s can now be closed, and their reset is more granular.
#1063
- Trainers can control whether collectors should be reset prior to
training. #1063
- Convenience constructor for `CollectStats` called
`with_autogenerated_stats`. #1063
### Internal Improvements
- `Collector`s rely less on state, the few stateful things are stored
explicitly instead of through a `.data` attribute. #1063
- Introduced a first iteration of a naming convention for vars in
`Collector`s. #1063
- Generally improved readability of Collector code and associated tests
(still quite some way to go). #1063
- Improved typing for `exploration_noise` and within Collector. #1063
### Breaking Changes
- Removed `.data` attribute from `Collector` and its child classes.
#1063
- Collectors no longer reset the environment on initialization. Instead,
the user might have to call `reset`
expicitly or pass `reset_before_collect=True` . #1063
- VectorEnvs now return an array of info-dicts on reset instead of a
list. #1063
- Fixed `iter(Batch(...)` which now behaves the same way as
`Batch(...).__iter__()`. Can be considered a bugfix. #1063
---------
Co-authored-by: Michael Panchenko <m.panchenko@appliedai.de>
Preparation for #914 and #920
Changes formatting to ruff and black. Remove python 3.8
## Additional Changes
- Removed flake8 dependencies
- Adjusted pre-commit. Now CI and Make use pre-commit, reducing the
duplication of linting calls
- Removed check-docstyle option (ruff is doing that)
- Merged format and lint. In CI the format-lint step fails if any
changes are done, so it fulfills the lint functionality.
---------
Co-authored-by: Jiayi Weng <jiayi@openai.com>
# Goals of the PR
The PR introduces **no changes to functionality**, apart from improved
input validation here and there. The main goals are to reduce some
complexity of the code, to improve types and IDE completions, and to
extend documentation and block comments where appropriate. Because of
the change to the trainer interfaces, many files are affected (more
details below), but still the overall changes are "small" in a certain
sense.
## Major Change 1 - BatchProtocol
**TL;DR:** One can now annotate which fields the batch is expected to
have on input params and which fields a returned batch has. Should be
useful for reading the code. getting meaningful IDE support, and
catching bugs with mypy. This annotation strategy will continue to work
if Batch is replaced by TensorDict or by something else.
**In more detail:** Batch itself has no fields and using it for
annotations is of limited informational power. Batches with fields are
not separate classes but instead instances of Batch directly, so there
is no type that could be used for annotation. Fortunately, python
`Protocol` is here for the rescue. With these changes we can now do
things like
```python
class ActionBatchProtocol(BatchProtocol):
logits: Sequence[Union[tuple, torch.Tensor]]
dist: torch.distributions.Distribution
act: torch.Tensor
state: Optional[torch.Tensor]
class RolloutBatchProtocol(BatchProtocol):
obs: torch.Tensor
obs_next: torch.Tensor
info: Dict[str, Any]
rew: torch.Tensor
terminated: torch.Tensor
truncated: torch.Tensor
class PGPolicy(BasePolicy):
...
def forward(
self,
batch: RolloutBatchProtocol,
state: Optional[Union[dict, Batch, np.ndarray]] = None,
**kwargs: Any,
) -> ActionBatchProtocol:
```
The IDE and mypy are now very helpful in finding errors and in
auto-completion, whereas before the tools couldn't assist in that at
all.
## Major Change 2 - remove duplication in trainer package
**TL;DR:** There was a lot of duplication between `BaseTrainer` and its
subclasses. Even worse, it was almost-duplication. There was also
interface fragmentation through things like `onpolicy_trainer`. Now this
duplication is gone and all downstream code was adjusted.
**In more detail:** Since this change affects a lot of code, I would
like to explain why I thought it to be necessary.
1. The subclasses of `BaseTrainer` just duplicated docstrings and
constructors. What's worse, they changed the order of args there, even
turning some kwargs of BaseTrainer into args. They also had the arg
`learning_type` which was passed as kwarg to the base class and was
unused there. This made things difficult to maintain, and in fact some
errors were already present in the duplicated docstrings.
2. The "functions" a la `onpolicy_trainer`, which just called the
`OnpolicyTrainer.run`, not only introduced interface fragmentation but
also completely obfuscated the docstring and interfaces. They themselves
had no dosctring and the interface was just `*args, **kwargs`, which
makes it impossible to understand what they do and which things can be
passed without reading their implementation, then reading the docstring
of the associated class, etc. Needless to say, mypy and IDEs provide no
support with such functions. Nevertheless, they were used everywhere in
the code-base. I didn't find the sacrifices in clarity and complexity
justified just for the sake of not having to write `.run()` after
instantiating a trainer.
3. The trainers are all very similar to each other. As for my
application I needed a new trainer, I wanted to understand their
structure. The similarity, however, was hard to discover since they were
all in separate modules and there was so much duplication. I kept
staring at the constructors for a while until I figured out that
essentially no changes to the superclass were introduced. Now they are
all in the same module and the similarities/differences between them are
much easier to grasp (in my opinion)
4. Because of (1), I had to manually change and check a lot of code,
which was very tedious and boring. This kind of work won't be necessary
in the future, since now IDEs can be used for changing signatures,
renaming args and kwargs, changing class names and so on.
I have some more reasons, but maybe the above ones are convincing
enough.
## Minor changes: improved input validation and types
I added input validation for things like `state` and `action_scaling`
(which only makes sense for continuous envs). After adding this, some
tests failed to pass this validation. There I added
`action_scaling=isinstance(env.action_space, Box)`, after which tests
were green. I don't know why the tests were green before, since action
scaling doesn't make sense for discrete actions. I guess some aspect was
not tested and didn't crash.
I also added Literal in some places, in particular for
`action_bound_method`. Now it is no longer allowed to pass an empty
string, instead one should pass `None`. Also here there is input
validation with clear error messages.
@Trinkle23897 The functional tests are green. I didn't want to fix the
formatting, since it will change in the next PR that will solve #914
anyway. I also found a whole bunch of code in `docs/_static`, which I
just deleted (shouldn't it be copied from the sources during docs build
instead of committed?). I also haven't adjusted the documentation yet,
which atm still mentions the trainers of the type
`onpolicy_trainer(...)` instead of `OnpolicyTrainer(...).run()`
## Breaking Changes
The adjustments to the trainer package introduce breaking changes as
duplicated interfaces are deleted. However, it should be very easy for
users to adjust to them
---------
Co-authored-by: Michael Panchenko <m.panchenko@appliedai.de>
Changes:
- Disclaimer in README
- Replaced all occurences of Gym with Gymnasium
- Removed code that is now dead since we no longer need to support the
old step API
- Updated type hints to only allow new step API
- Increased required version of envpool to support Gymnasium
- Increased required version of PettingZoo to support Gymnasium
- Updated `PettingZooEnv` to only use the new step API, removed hack to
also support old API
- I had to add some `# type: ignore` comments, due to new type hinting
in Gymnasium. I'm not that familiar with type hinting but I believe that
the issue is on the Gymnasium side and we are looking into it.
- Had to update `MyTestEnv` to support `options` kwarg
- Skip NNI tests because they still use OpenAI Gym
- Also allow `PettingZooEnv` in vector environment
- Updated doc page about ReplayBuffer to also talk about terminated and
truncated flags.
Still need to do:
- Update the Jupyter notebooks in docs
- Check the entire code base for more dead code (from compatibility
stuff)
- Check the reset functions of all environments/wrappers in code base to
make sure they use the `options` kwarg
- Someone might want to check test_env_finite.py
- Is it okay to allow `PettingZooEnv` in vector environments? Might need
to update docs?
## implementation
I implemented HER solely as a replay buffer. It is done by temporarily
directly re-writing transitions storage (`self._meta`) during the
`sample_indices()` call. The original transitions are cached and will be
restored at the beginning of the next sampling or when other methods is
called. This will make sure that. for example, n-step return calculation
can be done without altering the policy.
There is also a problem with the original indices sampling. The sampled
indices are not guaranteed to be from different episodes. So I decided
to perform re-writing based on the episode. This guarantees that the
sampled transitions from the same episode will have the same re-written
goal. This also make the re-writing ratio calculation slightly differ
from the paper, but it won't be too different if there are many episodes
in the buffer.
In the current commit, HER replay buffer only support 'future' strategy
and online sampling. This is the best of HER in term of performance and
memory efficiency.
I also add a few more convenient replay buffers
(`HERVectorReplayBuffer`, `HERReplayBufferManager`), test env
(`MyGoalEnv`), gym wrapper (`TruncatedAsTerminated`), unit tests, and a
simple example (examples/offline/fetch_her_ddpg.py).
## verification
I have added unit tests for almost everything I have implemented.
HER replay buffer was also tested using DDPG on [`FetchReach-v3`
env](https://github.com/Farama-Foundation/Gymnasium-Robotics). I used
default DDPG parameters from mujoco example and didn't tune anything
further to get this good result! (train script:
examples/offline/fetch_her_ddpg.py).
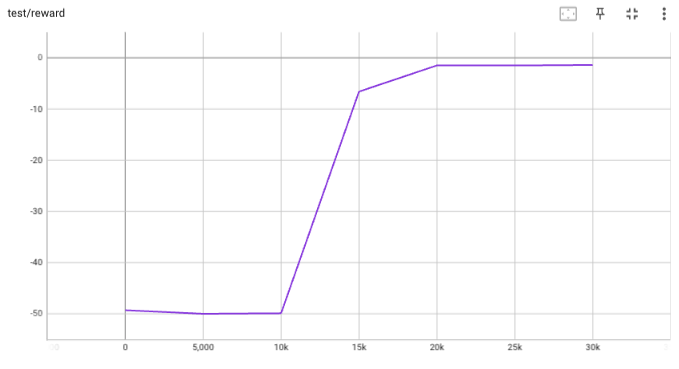
* Changes to support Gym 0.26.0
* Replace map by simpler list comprehension
* Use syntax that is compatible with python 3.7
* Format code
* Fix environment seeding in test environment, fix buffer_profile test
* Remove self.seed() from __init__
* Fix random number generation
* Fix throughput tests
* Fix tests
* Removed done field from Buffer, fixed throughput test, turned off wandb, fixed formatting, fixed type hints, allow preprocessing_fn with truncated and terminated arguments, updated docstrings
* fix lint
* fix
* fix import
* fix
* fix mypy
* pytest --ignore='test/3rd_party'
* Use correct step API in _SetAttrWrapper
* Format
* Fix mypy
* Format
* Fix pydocstyle.
Change the behavior of to_numpy and to_torch: from now on, dict is automatically converted to Batch and list is automatically converted to np.ndarray (if an error occurs, raise the exception instead of converting each element in the list).
This is the third PR of 6 commits mentioned in #274, which features refactor of Collector to fix#245. You can check #274 for more detail.
Things changed in this PR:
1. refactor collector to be more cleaner, split AsyncCollector to support asyncvenv;
2. change buffer.add api to add(batch, bffer_ids); add several types of buffer (VectorReplayBuffer, PrioritizedVectorReplayBuffer, etc.)
3. add policy.exploration_noise(act, batch) -> act
4. small change in BasePolicy.compute_*_returns
5. move reward_metric from collector to trainer
6. fix np.asanyarray issue (different version's numpy will result in different output)
7. flake8 maxlength=88
8. polish docs and fix test
Co-authored-by: n+e <trinkle23897@gmail.com>
This is the second commit of 6 commits mentioned in #274, which features minor refactor of ReplayBuffer and adding two new ReplayBuffer classes called CachedReplayBuffer and ReplayBufferManager. You can check #274 for more detail.
1. Add ReplayBufferManager (handle a list of buffers) and CachedReplayBuffer;
2. Make sure the reserved keys cannot be edited by methods like `buffer.done = xxx`;
3. Add `set_batch` method for manually choosing the batch the ReplayBuffer wants to handle;
4. Add `sample_index` method, same as `sample` but only return index instead of both index and batch data;
5. Add `prev` (one-step previous transition index), `next` (one-step next transition index) and `unfinished_index` (the last modified index whose done==False);
6. Separate `alloc_fn` method for allocating new memory for `self._meta` when a new `(key, value)` pair comes in;
7. Move buffer's documentation to `docs/tutorials/concepts.rst`.
Co-authored-by: n+e <trinkle23897@gmail.com>
Training FPS improvement (base commit is 94bfb32):
test_pdqn: 1660 (without numba) -> 1930
discrete/test_ppo: 5100 -> 5170
since nstep has little impact on overall performance, the unit test result is:
GAE: 4.1s -> 0.057s
nstep: 0.3s -> 0.15s (little improvement)
Others:
- fix a bug in ttt set_eps
- keep only sumtree in segment tree implementation
- dirty fix for asyncVenv check_id test
This PR aims to provide the script of Atari DQN setting:
- A speedrun of PongNoFrameskip-v4 (finished, about half an hour in i7-8750 + GTX1060 with 1M environment steps)
- A general script for all atari game
Since we use multiple env for simulation, the result is slightly different from the original paper, but consider to be acceptable.
It also adds another parameter save_only_last_obs for replay buffer in order to save the memory.
Co-authored-by: Trinkle23897 <463003665@qq.com>
1. add policy.eval() in all test scripts' "watch performance"
2. remove dict return support for collector preprocess_fn
3. add `__contains__` and `pop` in batch: `key in batch`, `batch.pop(key, deft)`
4. exact n_episode for a list of n_episode limitation and save fake data in cache_buffer when self.buffer is None (#184)
5. fix tensorboard logging: h-axis stands for env step instead of gradient step; add test results into tensorboard
6. add test_returns (both GAE and nstep)
7. change the type-checking order in batch.py and converter.py in order to meet the most often case first
8. fix shape inconsistency for torch.Tensor in replay buffer
9. remove `**kwargs` in ReplayBuffer
10. remove default value in batch.split() and add merge_last argument (#185)
11. improve nstep efficiency
12. add max_batchsize in onpolicy algorithms
13. potential bugfix for subproc.wait
14. fix RecurrentActorProb
15. improve the code-coverage (from 90% to 95%) and remove the dead code
16. fix some incorrect type annotation
The above improvement also increases the training FPS: on my computer, the previous version is only ~1800 FPS and after that, it can reach ~2050 (faster than v0.2.4.post1).
* bugfix for update with empty buffer; remove duplicate variable _weight_sum in PrioritizedReplayBuffer
* point out that ListReplayBuffer cannot be sampled
* remove useless _amortization_counter variable
* Use lower-level API to reduce overhead.
* Further improvements.
* Buffer _add_to_buffer improvement.
* Do not use _data field to store Batch data to avoid overhead. Add back _meta field in Buffer.
* Restore metadata attribute to store batch in Buffer.
* Move out nested methods.
* Update try/catch instead of actual check to efficiency.
* Remove unsed branches for efficiency.
* Use np.array over list when possible for efficiency.
* Final performance improvement.
* Add unit tests for Batch size method.
* Add missing stack unit tests.
* Enforce Buffer initialization to zero.
Co-authored-by: Alexis Duburcq <alexis.duburcq@wandercraft.eu>